Automasking Guide CASA 5: Difference between revisions
Line 125: | Line 125: | ||
=== Base Parameters === | === Base Parameters === | ||
To figure out what automasking | To figure out what automasking parameter values we should use for this data, we run [https://safe.nrao.edu/wiki/bin/view/ALMA/GetBaselineStats au.getBaselineStats] which will tell us the "b75" value for our data and, thus, whether our data is "long" or "short" baseline. | ||
<source lang="python"> | <source lang="python"> | ||
CASA | #In CASA | ||
au.getBaselineStats('sis14_twhya_calibrated_flagged.ms.contsub') | |||
</source> | |||
Found 325 baselines (26 stations) | Found 325 baselines (26 stations) | ||
Unprojected lengths: min=15.063874, max=374.719747, rms=162.602204 m | Unprojected lengths: min=15.063874, max=374.719747, rms=162.602204 m | ||
number=325, min=15.06m, max=374.72m, median=139.34m, mean=142.78m, std=77.81m | number=325, min=15.06m, max=374.72m, median=139.34m, mean=142.78m, std=77.81m | ||
20%ile=69.4m 25%ile=79.0m, 30%ile=87.6m, 75%ile=197.4m, 90%ile=250.2m | 20%ile=69.4m 25%ile=79.0m, 30%ile=87.6m, 75%ile=197.4m, 90%ile=250.2m | ||
We can see from the output above that b75 (75%ile) = 194.4m, which puts us solidly in the "short" baseline category. Therefore, here we use the “standard”, base automasking values that the pipeline uses for 12m short baseline data from the table above. This will give us a mask to compare our testing against and will be displayed as black contours in all of the following figures. | We can see from the output above that b75 (75%ile) = 194.4m, which puts us solidly in the "short" baseline category. Therefore, here we use the “standard”, base automasking values that the pipeline uses for 12m short baseline data from the table above. This will give us a mask to compare our testing against and will be displayed as black contours in all of the following figures. |
Revision as of 19:01, 2 May 2018
UNDER CONSTRUCTION
Introduction
Starting with CASA 5.1, a new algorithm called ‘auto-multithresh’ has been incorporated into tclean to automatically mask regions during the cleaning process. This algorithm is intended to mimic what an experienced user would do when manually masking images and can be used by setting the usemask option in tclean to ’auto-multithresh’.
A full description of the auto-multithresh algorithm is given in the CASA documentation. Here we briefly describe the fundamental features of the algorithm. A key feature of the auto-multithresh algorithm is that it operates on the residual image at the beginning of every minor cycle, so that the mask updates as the clean progresses. First, the algorithm identifies regions that are either above a signal-to-noise limit or a sidelobe level (as defined by the user), whichever is higher. If the mask regions are smaller than some fraction of the beam, they are removed (“pruned”) to avoid including spurious noise peaks in the masked region. If there is extended low signal to noise emission surrounding the initial mask then this emission is included in the mask by expanding the noise/sidelobe mask into the low signal-to-noise regions using a process called binary dilation. Finally, the mask is expanded to include a buffer region around the masked emission by convolving the mask by a Gaussian and then retaining only regions that are above n% of the peak in the smoothed mask. Absorption regions can also be masked using a method similar to that for the initial threshold mask, but are not pruned or extended into low signal-to noise regions. Note that for cubes, the algorithm masks each channel independently.
Parameters
Most of the parameters for the auto-multithresh algorithm are defined in terms of fundamental image properties (e.g., beam size, single-to-noise, etc) rather than exact numerical values (e.g., 3”, 6 mJy/beam). This feature allows the same set of parameters to be used for multiple images. The auto-multithresh algorithm calculates the numerical value of each parameter internally using the noise derived from the residual image itself (via the median absolute deviation) and the beam size and sidelobe level supplied by tclean internally.
The five primary auto-multithresh parameters control what thresholds the algorithm uses and how regions are removed from the mask via pruning.
- Noisethreshold (type: double) -- controls the signal-to-noise threshold above which significant emission is masked during the initial round of mask creation. Note that either noisethreshold or sidelobethreshold is used depending on which threshold is higher.
- Sidelobethreshold (type: double) -- controls the signal-to-noise threshold above which significant emission is masked during the initial round of mask creation. Note that either noisethreshold or sidelobethreshold is used depending on which threshold is higher.
- Minbeamfrac (type: double) -- controls the minimum size a region must be to be considered real emission in terms of fractions of the beam size. Any masks smaller than this will be removed before the next round of cleaning. Note that this parameter operates on both the initial threshold mask and the low signal-to-noise mask.
- Lownoisethreshold (type: double) -- controls the expansion of the initial mask (which is determined by noisethreshold or sidelobethreshold) into low signal-to-noise regions.
- Negativethreshold (type: double) -- controls the signal-to-noise threshold for absorption features to be masked. Note that any absorption features that are masked are not pruned or expanded into low signal-to-noise regions.
Auto-multithresh has several additional parameters that usually do not need to be changed from their default values:
- Growiterations (type: int) -- controls the number of iterations that binary dilation performs. Lowering this value can reduce the computational time needed to expand the mask into low signal-to-noise regions at the expense of potentially not expanding the mask all the way to its edges. A value between 75 and 100 is usually adequate.
- Smoothfactor (type: double) -- controls how much to smooth the initial mask. We do not recommend changing this from its default value.
- Cutthreshold (type: double) -- controls what regions of the smoothed mask are retained to form the final mask. We do not recommend changing this from its default value.
The default automasking parameters in tclean have been set to values appropriate for the ALMA 12m array in its more compact configurations (i.e. not long baseline data). These values depend on the PSF (i.e., uv-coverage) of the data. Data with PSFs significantly different than the fiducial ALMA 12m arrays (e.g. 7m data) will likely need the auto-multithresh parameters to be modified in order to achieve the best mask. The default pipeline parameters presented here (Table below) have been extensively tested and most likely will work for your data set, although you may wish to optimize them for your particular imaging case. Data from other instruments (e.g., VLA, ATCA, etc) has been shown to work with this algorithm in limited testing, but the parameter values may need to be optimized.
Here are some guidelines for optimizing auto-multithresh parameters:
- Start with the pipeline parameters for the data with the most similar parameters to your data set. When in doubt, choose the default short baseline 12m parameters.
- For cubes, use a small (~10 channel) cube with representative emission as a test case to avoid long run times.
- Change one parameter at a time and keep track of your previous images so that you can directly compare different runs.
- Inspect the log output. The ‘auto-multithresh’ algorithm reports extensive information to the logger on what it is doing including what thresholds are being used, how many regions are being pruned, etc.
- Save the masks for each major cycle. To save the intermediate masks, type the following on the casa command line: os.environ['SAVE_ALL_AUTOMASKS']="true". Note that you can also inspect the intermediate masks by setting interactive=True. However, the resulting masks will NOT necessarily be the same as masks generated with interactive=False because how often the minor cycle is triggered can be different in the two cases.
Table of Standard Values
The values presented in this table are the "standard" values that the pipeline uses when it performs automasking and have been extensively tested. As for combined data (the last row of the table), these values are listed as tentative and should serve as a starting point for your analysis and could be dependent on the type of emission you have.
Array | sidelobethreshold | noisethreshold | minbeamfrac | lownoisethreshold | negativethreshold |
12m (short) b75<300m | 2.0 | 4.25 | 0.3 | 1.5 | 0.0 (continuum)/15.0 (line) |
12m (long) b75>300m | 3.0 | 5.0 | 0.3 | 1.5 | 0.0 (continuum)/7.0 (line) |
7m (continnum/line) | 1.25 | 5.0 | 0.1 | 2.0 | 0.0 |
12m + 7m combined TENTATIVE | 2.0 | 4.25 | 0.3 | 1.5 | 0.0 |
The term “b75” refers to the 75th percentile of baselines. You can find this value in the weblog by going to the antenna configuration page and clicking on the “baselines” tab, the table lists the baseline length in increasing distance and the percentile. If you don’t have a weblog for your data, you may use the Analysis Utilities task au.getBaselineStats. You can obtain the Analysis Utilities tasks by following these instructions: https://casaguides.nrao.edu/index.php/Analysis_Utilities
Below, we provide some examples of how changing the input parameters changes the resulting mask.
Simple Examples of the Tclean Automasking Parameters and "What Can Go Wrong"
This section should serve as a guide to help you gain a more intuitive understanding of how the automasking algorithm works and understand better what each parameter does during the automasking process. Additionally, we will show you what can happen when you “over-tune” a parameter and end up masking noise features or not masking real emission.
To demonstrate the use of the automasking parameters, we will be using the TW Hydra dataset which is a small dataset from the “First Look” CASA Guides and is available here: https://bulk.cv.nrao.edu/almadata/sciver/TWHya/sis14_twhya_calibrated_flagged.ms.contsub.tgz
This file is the calibrated, continuum subtracted Band 7 data. Untar the file and you should see a file named sis14_twhya_calibrated_flagged.ms.contsub. Almost all of the values used in our tclean commands remain constant throughout this section and are explained and taken from the First Look at Spectral Line Imaging CASA Guide. The main difference is that we are using the usemask=’auto-multithresh’ parameter to use the automasking feature and will be adjusting the auto-masking subparameters to highlight their use.
Make a Dirty Cube
First we make a dirty cube to figure out the value for threshold and get an idea of what the emission/noise looks like.
#In CASA
tclean(vis='sis14_twhya_calibrated_flagged.ms.contsub',
imagename='twhya_dirtycube',
field='0',
spw='0',
specmode='cube',
nchan=15,
start='0.0km/s',
width='0.5km/s',
outframe='LSRK',
restfreq='372.67249GHz',
deconvolver='hogbom',
gridder='standard',
imsize=[250,250],
cell='0.08arcsec',
weighting='briggs',
robust=0.5,
restoringbeam='common',
interactive=False,
chanchunks=-1,
niter=100000,
threshold=’0.0Jy’,
# Automasking Parameters below this line
usemask='auto-multithresh’,
sidelobethreshold=2.0,
noisethreshold=4.25,
lownoisethreshold=1.5,
minbeamfrac=0.3,
growiterations=75,
negativethreshold=15.0)
Using the viewer and selecting a region in a line free channel (I used channel 3) we find that the RMS in a line free channel is 1.78e-2 Jy/beam. To set our threshold for cleaning, we set it to twice the RMS so threshold= 2*RMS = 0.0374 Jy.
Base Parameters
To figure out what automasking parameter values we should use for this data, we run au.getBaselineStats which will tell us the "b75" value for our data and, thus, whether our data is "long" or "short" baseline.
#In CASA
au.getBaselineStats('sis14_twhya_calibrated_flagged.ms.contsub')
Found 325 baselines (26 stations) Unprojected lengths: min=15.063874, max=374.719747, rms=162.602204 m number=325, min=15.06m, max=374.72m, median=139.34m, mean=142.78m, std=77.81m 20%ile=69.4m 25%ile=79.0m, 30%ile=87.6m, 75%ile=197.4m, 90%ile=250.2m
We can see from the output above that b75 (75%ile) = 194.4m, which puts us solidly in the "short" baseline category. Therefore, here we use the “standard”, base automasking values that the pipeline uses for 12m short baseline data from the table above. This will give us a mask to compare our testing against and will be displayed as black contours in all of the following figures.
#In CASA
os.system('rm -rf twhya_base_params.*')
tclean(vis='sis14_twhya_calibrated_flagged.ms.contsub',
imagename='twhya_base_params',
field='0',
spw='0',
specmode='cube',
nchan=15,
start='0.0km/s',
width='0.5km/s',
outframe='LSRK',
restfreq='372.67249GHz',
deconvolver='hogbom',
gridder='standard',
imsize=[250,250],
cell='0.08arcsec',
weighting='briggs',
robust=0.5,
restoringbeam='common',
interactive=False,
chanchunks=-1,
niter=100000,
threshold=’0.0356Jy’,
usemask='auto-multithresh’,
sidelobethreshold=2.0,
noisethreshold=4.25,
lownoisethreshold=1.5,
minbeamfrac=0.3,
growiterations=75,
negativethreshold=15.0)
Turning Down noisethreshold
First we start by turning down the value for noisethreshold to something like 1.5.
<figure id="small_noisethreshold.png">
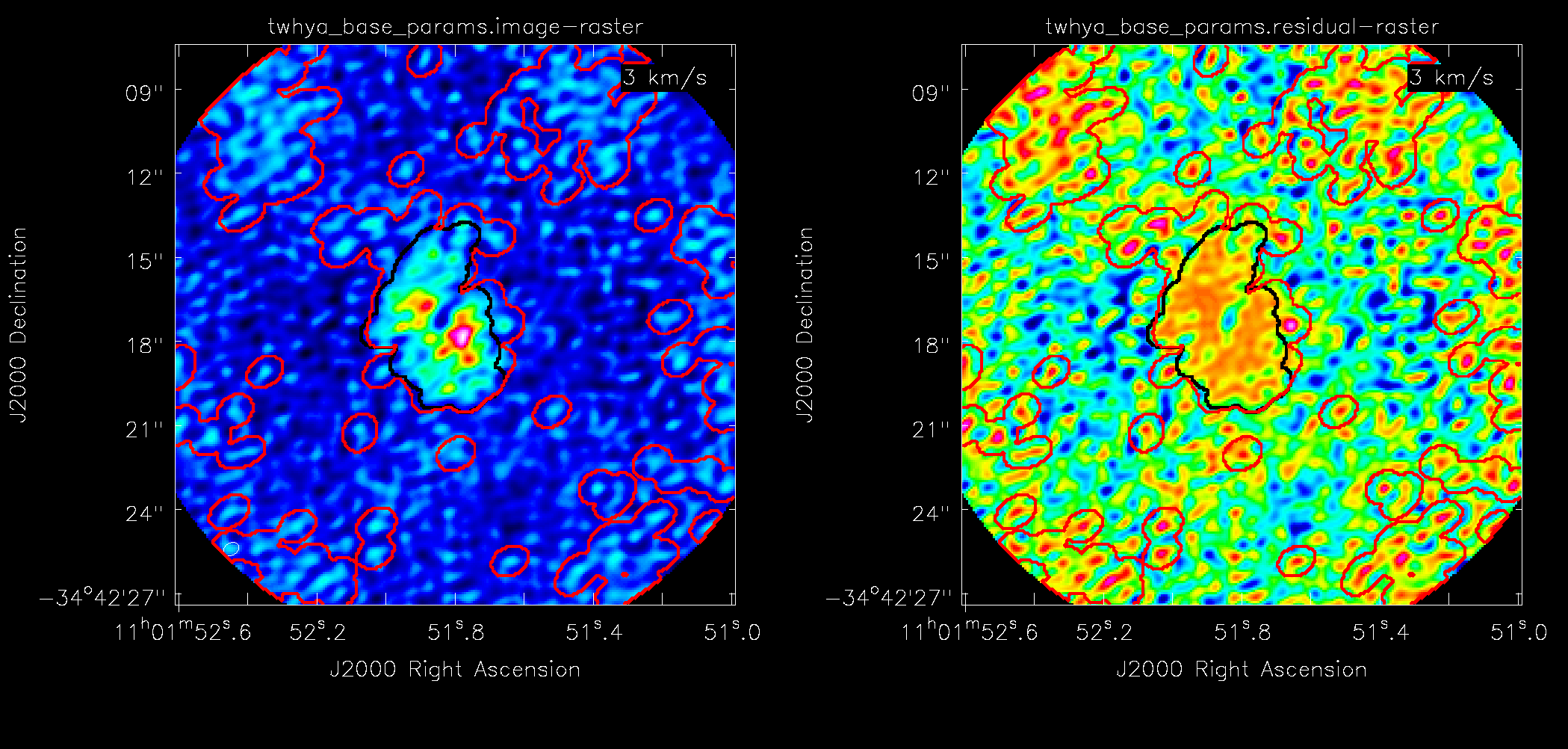
</figure>
#In CASA
os.system('rm -rf twhya_lownoise_params.*')
tclean(vis='sis14_twhya_calibrated_flagged.ms.contsub',
imagename='twhya_lownoise_params',
field='0',
spw='0',
specmode='cube',
nchan=15,
start='0.0km/s',
width='0.5km/s',
outframe='LSRK',
restfreq='372.67249GHz',
deconvolver='hogbom',
gridder='standard',
imsize=[250,250],
cell='0.08arcsec',
weighting='briggs',
robust=0.5,
restoringbeam='common',
interactive=False,
chanchunks=-1,
niter=100000,
threshold=’0.0356Jy’,
usemask='auto-multithresh’,
sidelobethreshold=2.0,
noisethreshold=1.5,
lownoisethreshold=1.5,
minbeamfrac=0.3,
growiterations=75,
negativethreshold=15.0)
What we see is that since noisethreshold is too low, sidelobetheshold is used instead to draw the initial masks. You can see this in the logger while the automasking is working, tclean reports which threshold is being used to find maskable emission in the image.
----------------------------------------------------------- Run Major Cycle 1 ------------------------------------- Found full images : 1 Found part images : 0 [twhya_lownoise_params] Peak residual (max,min) over full image : (0.149852,-0.0818088) [twhya_lownoise_params] Total Model Flux : 0 [twhya_lownoise_params] Setting up an auto-mask within PB mask limit Generating AutoMask SidelobeLevel = 0.219842 prune size=13.5358(minbeamfrac=0.3 * beampix=45.1195) rms from MAD (mads*1.4826)= [0.0194964, 0.018565, 0.0193204, 0.0185711, 0.0192612, 0.0224527, 0.0256428, 0.0212457, 0.0176657, 0.0175954, 0.0178157, 0.0176049, 0.0176475, 0.0178578, 0.0171668] Using sidelobe threshold for chan 0 threshold=0.0308191 Using sidelobe threshold for chan 1 threshold=0.0304624 Using sidelobe threshold for chan 2 threshold=0.0314644 Using sidelobe threshold for chan 3 threshold=0.0307041 Using sidelobe threshold for chan 4 threshold=0.0380167 Using sidelobe threshold for chan 5 threshold=0.0585222 Using sidelobe threshold for chan 6 threshold=0.0658874 Using sidelobe threshold for chan 7 threshold=0.0513938 Using sidelobe threshold for chan 8 threshold=0.0326665 Using sidelobe threshold for chan 9 threshold=0.0289218 Using sidelobe threshold for chan 10 threshold=0.0288309 Using sidelobe threshold for chan 11 threshold=0.028183 Using sidelobe threshold for chan 12 threshold=0.0299507 Using sidelobe threshold for chan 13 threshold=0.0337656 Using sidelobe threshold for chan 14 threshold=0.0296112
In later cycles, we can see that some channels switch to using the noisethreshold. Which threshold tclean uses can differ per channel and can change between major cycles as clean progresses. In the image below we see that fainter emission gets picked up by automasking when we reduce the noisethreshold value (as expected) but clearly noise spikes are being picked up in both line and line-free channels.
As well as choosing between noisethreshold and sidelobethreshold, automasking additionally chooses between lownoisethreshold and sidelobethreshold during the second round of masking when the mask is grown into low signal-to-noise regions. The actual logic and values that are used for each threshold are the following.
lowNoiseThresholdValue = lowNoiseThreshold * rms in residual image
sidelobeThresholdValue = sidelobeThreshold * sidelobeLevel * peak in residual image
noiseThresholdValue = noiseThreshold * rms in residual image
Where the sidelobeLevel is calculated by tclean and reported in the logger (you can see this in the example logger output above). The value that is used in the first round of masking is
max(sidelobeThresholdValue,noiseThresholdValue).
Then, for the second round of masking where the mask is expanded into low signal-to-noise regions the threshold that is used is determined by
max(sidelobeThresholdValue,lowNoiseThresholdValue).
In practice, the sidlobethreshold often sets the limit before noisethreshold because the sidelobe emission is often larger than the random noise in the image. Also, if the sidelobethreshold is used to set the initial mask, then the mask will not be grown at all because it will also be larger than the lownoisetheshold.
Turning Down sidelobethreshold
Now we turn sidelobethreshold down to see what impact this has on the masks that are created. Note that because of what we found out above about how masks are drawn during each step of the automasking process, we need to also turn down either noisethreshold or lownoisethreshold to guarantee that the sidelobethreshold is used by the algorithm during either the initial step or the secondary growing of the masks step.
<figure id="small_sidelobethreshold.png">
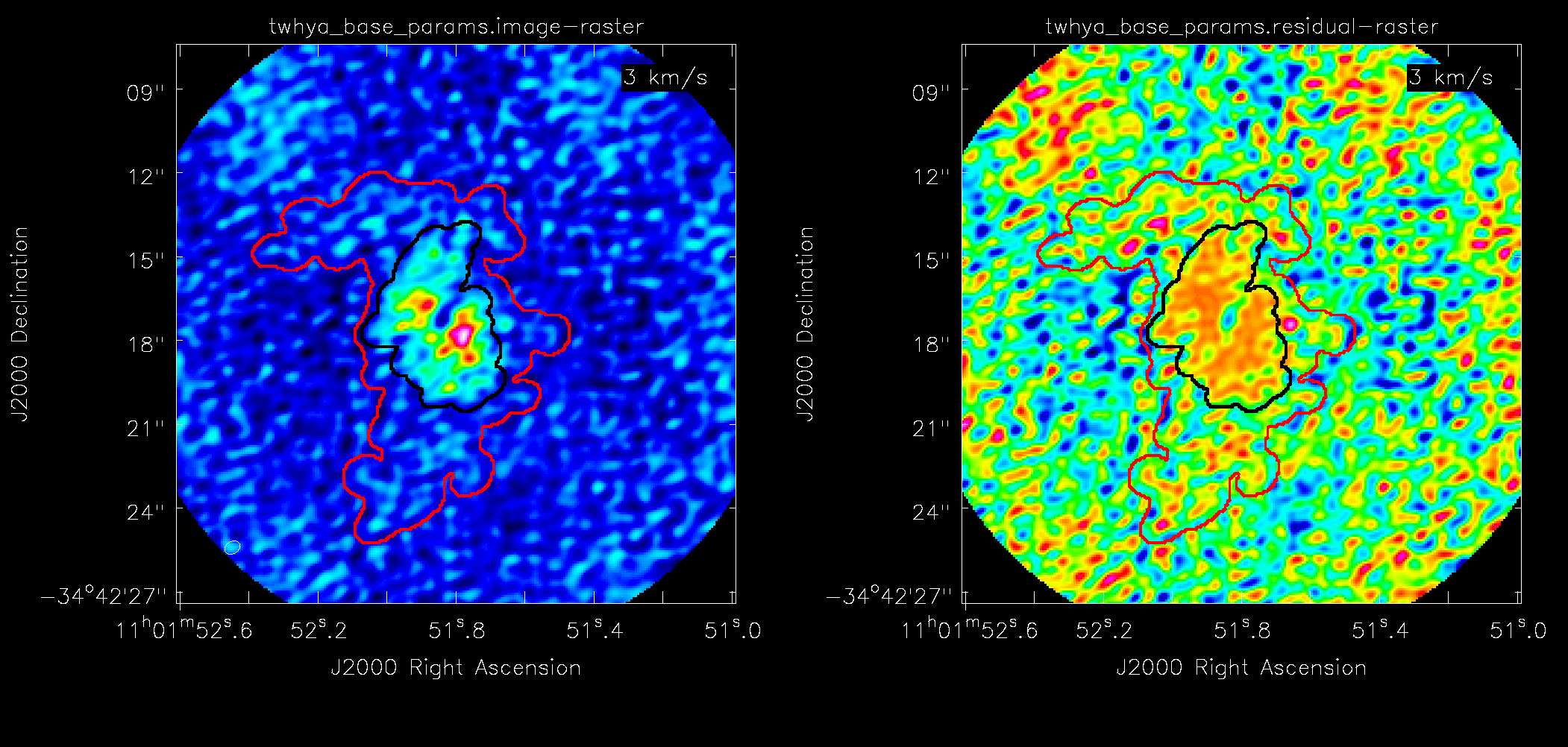
</figure>
#In CASA
os.system('rm -rf twhya_lowsidelobe_params.*')
tclean(vis='sis14_twhya_calibrated_flagged.ms.contsub',
imagename='twhya_lowsidelobe_params',
field='0',
spw='0',
specmode='cube',
nchan=15,
start='0.0km/s',
width='0.5km/s',
outframe='LSRK',
restfreq='372.67249GHz',
deconvolver='hogbom',
gridder='standard',
imsize=[250,250],
cell='0.08arcsec',
weighting='briggs',
robust=0.5,
restoringbeam='common',
interactive=False,
chanchunks=-1,
niter=100000,
threshold=’0.0356Jy’,
usemask='auto-multithresh’,
sidelobethreshold=1.0,
noisethreshold=4.25,
lownoisethreshold=0.5,
minbeamfrac=0.3,
growiterations=75,
negativethreshold=15.0)
In Figure 2 we see that by turning down sidelobethreshold makes a mask that covers a much larger region and clearly covers areas of the image that do not contain real emission.
Turning down noisethreshold With No Pruning (i.e. minbeamfrac=0.0)
Now we turn down noisethreshold but also turn off pruning by setting minbeamfrac to 0.0 so that no regions are removed from the mask.
<figure id="small_noisethreshold_nopruning.png">

</figure>
#In CASA
os.system('rm -rf twhya_lownoise_noprune_params.*')
tclean(vis='sis14_twhya_calibrated_flagged.ms.contsub',
imagename='twhya_lownoise_noprune_params',
field='0',
spw='0',
specmode='cube',
nchan=15,
start='0.0km/s',
width='0.5km/s',
outframe='LSRK',
restfreq='372.67249GHz',
deconvolver='hogbom',
gridder='standard',
imsize=[250,250],
cell='0.08arcsec',
weighting='briggs',
robust=0.5,
restoringbeam='common',
interactive=False,
chanchunks=-1,
niter=100000,
threshold=’0.0356Jy’,
usemask='auto-multithresh’,
sidelobethreshold=2.0,
noisethreshold=3.5,
lownoisethreshold=1.5,
minbeamfrac=0.0,
growiterations=75,
negativethreshold=15.0)
In Figure 3 we show a line free channel. We see that using the base parameters, no masks were created but with a small noisethreshold value and no pruning, noise spikes are picked up and masked by the algorithm.
Base Parameters With Over Pruning
Next, we set minbeamfrac to 1.0 so that only masks smaller than the beam will get pruned.
#In CASA
os.system('rm -rf twhya_highminbeamfrac_params.*')
tclean(vis='sis14_twhya_calibrated_flagged.ms.contsub',
imagename='twhya_highminbeamfrac_params',
field='0',
spw='0',
specmode='cube',
nchan=15,
start='0.0km/s',
width='0.5km/s',
outframe='LSRK',
restfreq='372.67249GHz',
deconvolver='hogbom',
gridder='standard',
imsize=[250,250],
cell='0.08arcsec',
weighting='briggs',
robust=0.5,
restoringbeam='common',
interactive=False,
chanchunks=-1,
niter=100000,
threshold=’0.0356Jy’,
usemask='auto-multithresh’,
sidelobethreshold=2.0,
noisethreshold=4.25,
lownoisethreshold=1.5,
minbeamfrac=1.0,
growiterations=75,
negativethreshold=15.0)
Masks were found but then immediately removed and a dirty cube was generated! Whomp.
CASA$ WARN 3 of 15 channels had all regions removed by pruning. Try decreasing minbeamfrac to remove fewer regions. CASA$ WARN Restoring with an empty model image. Only residuals will be processed to form the output restored image.
This is because automasking initially finds regions of pixels with high signal-to-noise which are often not large patches of emission and smaller than the beam size. Thus with minbeamfrac set to 1.0, all the masks are pruned.
Turning Down lownoisethreshold
Finally, we lower the lownoisethreshold value to try and grow the mask into regions of lower signal-to-noise during the binary dilation stage.
#In CASA
os.system('rm -rf twhya_lowlownoise_params.*')
tclean(vis='sis14_twhya_calibrated_flagged.ms.contsub',
imagename='twhya_lowlownoise_params',
field='0',
spw='0',
specmode='cube',
nchan=15,
start='0.0km/s',
width='0.5km/s',
outframe='LSRK',
restfreq='372.67249GHz',
deconvolver='hogbom',
gridder='standard',
imsize=[250,250],
cell='0.08arcsec',
weighting='briggs',
robust=0.5,
restoringbeam='common',
interactive=False,
chanchunks=-1,
niter=100000,
threshold=’0.0356Jy’,
usemask='auto-multithresh’,
sidelobethreshold=2.0,
noisethreshold=4.25,
lownoisethreshold=0.5,
minbeamfrac=0.3,
growiterations=75,
negativethreshold=15.0)
Hmm... this offered absolutely no change compared to the base values even if we try to make the lownoisethreshold very, very small (i.e. something like 0.0001). This is due to the fact that, as we saw above, if lownoisethreshold is set lower than sidelobethreshold, then sidelobethreshold becomes the lowest cap. Therefore, if the sidelobeThresholdValue is used to set the mask and is larger than lowNoiseThresholdValue, then the mask is not grown at all. This effectively attempts to act as a “fail-safe” to ensure that overmasking does not occur accidentally by setting one of the thresholds very small. However, for demonstration purposes, we can force it to use a small lownoisethreshold by also setting the sidelobethreshold very small.
<figure id="Small_lownoisethreshold.png">
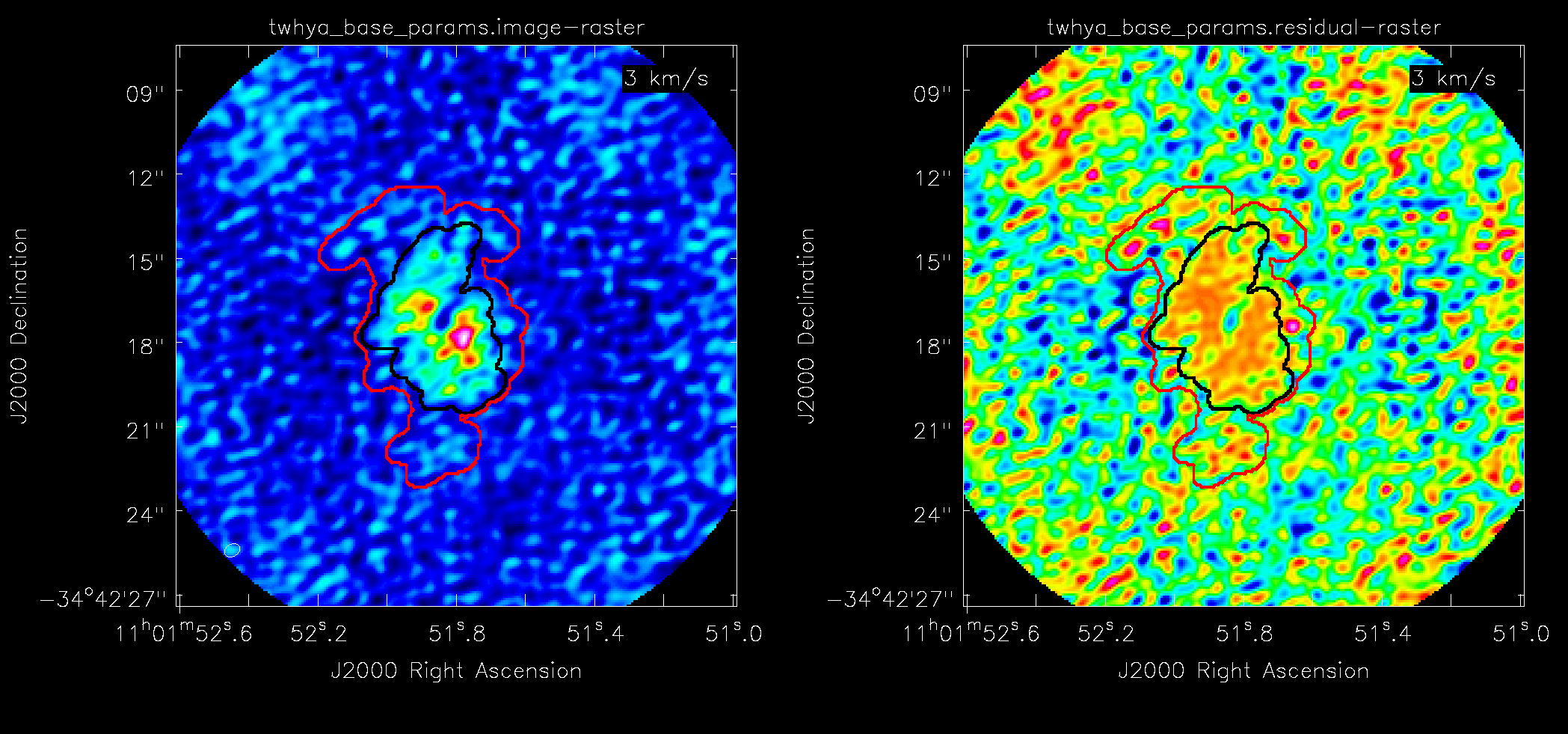
</figure>
os.system('rm -rf twhya_low_lownoise+sidelobe_params.*')
tclean(vis='sis14_twhya_calibrated_flagged.ms.contsub',
imagename='twhya_low_lownoise+sidelobe_params',
field='0',
spw='0',
specmode='cube',
nchan=15,
start='0.0km/s',
width='0.5km/s',
outframe='LSRK',
restfreq='372.67249GHz',
deconvolver='hogbom',
gridder='standard',
imsize=[250,250],
cell='0.08arcsec',
weighting='briggs',
robust=0.5,
restoringbeam='common',
interactive=False,
chanchunks=-1,
niter=100000,
threshold=’0.0356Jy’,
usemask='auto-multithresh’,
sidelobethreshold=0.5,
noisethreshold=4.25,
lownoisethreshold=1.0,
minbeamfrac=0.3,
growiterations=75,
negativethreshold=15.0)
We can see the result of this in Figure 4 which shows a much more extended mask that covers some nearby emission but extends much further into noise structures.