Baseband Stitching
Overview
In order to maintain constant rms noise across an observed frequency range, one may "overlap" EVLA basebands, offsetting one by 0.5 times the subband width. This way, the decreased sensitivity at subband edges can be edited out, and these frequency ranges replaced with data from the other baseband.
The contributed task stitch can be used to make this process simpler. Given a basic set of inputs, it will select channel ranges for each spectral window, which can then be used to run split to create a new measurement set (MS) (stitch can also run split, further simplifying things).
Here, we demonstrate the use of the stitch task. For information on reducing the data after running stitch, see the other CASA Guides EVLA tutorials — the data can be treated in the standard way.
Obtaining and installing stitch
First, you will need to download two files that are needed to build the task:
File:Task stitch.py File:Stitch.xml
Because of a quirk in the way Wiki stores files, you need to rename these to no longer have capitalized first letters, task_stitch.py and stitch.xml. Next, run buildmytasks to build the needed files for the task. This can either be done in CASA, or in a UNIX shell. You must be inside the directory where you have saved the files.
# In CASA
!buildmytasks
or:
# In the shell
buildmytasks
Once this completes, import the task into CASA:
# In CASA
execfile('mytasks.py')
You should now be able to look at the help and parameter listings of stitch:
# In CASA
inp stitch
and
# In CASA
help stitch
Inspecting data and choosing channel range
For the purposes of this guide, we are using EVLA test data that was taken in the overlapping subband mode. This dataset may be found in the EVLA Data Archive; the archive file id is TVER0002_sb2557689_1.55517.018916574074. Here, we have imported it to an MS called overlapSubband.ms.
To start with, we have a look at the data with listobs:
# In CASA:
listobs('overlapSubband.ms')
The most important part of the output is the spectral window information:
Spectral Windows: (18 unique spectral windows and 1 unique polarization setups)
SpwID #Chans Frame Ch1(MHz) ChanWid(kHz) TotBW(kHz) Corrs 0 64 TOPO 4736 2000 128000 RR RL LR LL 1 64 TOPO 7836 2000 128000 RR RL LR LL 2 64 TOPO 4488 2000 128000 RR RL LR LL 3 64 TOPO 4616 2000 128000 RR RL LR LL 4 64 TOPO 4744 2000 128000 RR RL LR LL 5 64 TOPO 4872 2000 128000 RR RL LR LL 6 64 TOPO 5000 2000 128000 RR RL LR LL 7 64 TOPO 5128 2000 128000 RR RL LR LL 8 64 TOPO 5256 2000 128000 RR RL LR LL 9 64 TOPO 5384 2000 128000 RR RL LR LL 10 64 TOPO 4552 2000 128000 RR RL LR LL 11 64 TOPO 4680 2000 128000 RR RL LR LL 12 64 TOPO 4808 2000 128000 RR RL LR LL 13 64 TOPO 4936 2000 128000 RR RL LR LL 14 64 TOPO 5064 2000 128000 RR RL LR LL 15 64 TOPO 5192 2000 128000 RR RL LR LL 16 64 TOPO 5320 2000 128000 RR RL LR LL 17 64 TOPO 5448 2000 128000 RR RL LR LL
For this observation, we can see that spectral windows 0 and 1 are used for the "dummy" scans, then science data were taken in SPW 2-17. Furthermore, SPW 2-9 are contiguous and in the first baseband, then the frequency jumps back down again, and SPW 10-17 (second baseband) are shifted by half a SPW up in frequency relative to the first baseband. We can also see this by plotting the data:
# In CASA:
plotms(vis='overlapSubband.ms', field='1', antenna='ea21', xaxis='freq',
yaxis='amp', coloraxis='spw', correlation='rr', avgtime='30s')
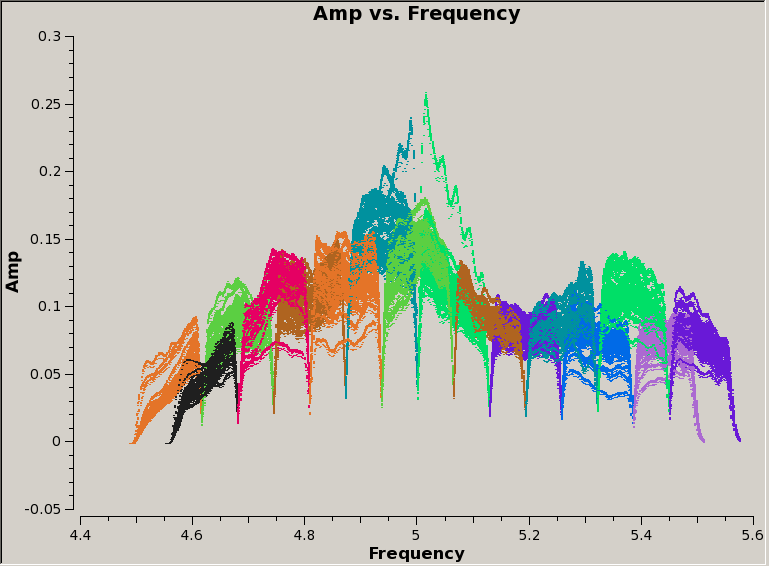
Note that we're only plotting data for one field, antenna, and correlation, and averaging in time by 30 seconds. Also, we're coloring the data points by their spectral window, to show visually the overlap in frequency between the two basebands.