VLA CASA Flagging-CASA4.5.2: Difference between revisions
No edit summary |
|||
Line 20: | Line 20: | ||
'''importasdm(asdm='AB1345_sb1800808_1.55431.004049953706', vis='SNR_G55.ms', process_flags=True, tbuff=1.5, applyflags=False, outfile='SNR_G55.ms.onlineflags.txt', flagbackup=False)''' | '''importasdm(asdm='AB1345_sb1800808_1.55431.004049953706', vis='SNR_G55.ms', process_flags=True, tbuff=1.5, applyflags=False, outfile='SNR_G55.ms.onlineflags.txt', flagbackup=False)''' | ||
process_flags = True: This parameter controls the creation of online flags from the Flag.xml SDM table. It will create online flags in the FLAG_CMD sub-table within the MS (more on this later). | process_flags=True: This parameter controls the creation of online flags from the Flag.xml SDM table. | ||
tbuff = 1.5: This parameter adds a time "buffer" padding to the flags in both directions to deal with timing mismatches. This is important for JVLA data taken before April 2011. This value should be set to 1.5x integration time. This particular observation had 1 second integrations. (CASA Cookbook section 2.2.2 and 3.5.1.3) | It will create online flags in the FLAG_CMD sub-table within the MS (more on this later).<br /> | ||
applyflags = False: We will apply these flags later in the tutorial. | tbuff=1.5: This parameter adds a time "buffer" padding to the flags in both directions to deal with timing mismatches. | ||
outfile = 'SNR_G55.ms.onlineflags.txt': This will create a text file with a list of online flags that can be applied. Created just for convenience, and won't be used during the tutorial. | This is important for JVLA data taken before April 2011. This value should be set to 1.5x integration time. | ||
This particular observation had 1 second integrations. (CASA Cookbook section 2.2.2 and 3.5.1.3)<br /> | |||
applyflags=False: We will apply these flags later in the tutorial.<br /> | |||
outfile='SNR_G55.ms.onlineflags.txt': This will create a text file with a list of online flags that can be applied. | |||
Created just for convenience, and won't be used during the tutorial. | |||
Split and time-averaged using the split task. For CASA 4.5.3 we use the split2() implementation: | Split and time-averaged using the split task. For CASA 4.5.3 we use the split2() implementation: | ||
Line 29: | Line 33: | ||
'''split2(vis='SNR_G55.ms', outputvis='SNR_G55_10s.ms', field='3~5', spw='4~5,7~8', antenna='!ea06,ea17,ea20,ea26', datacolumn='data', keepflags=False, timebin='10s')''' | '''split2(vis='SNR_G55.ms', outputvis='SNR_G55_10s.ms', field='3~5', spw='4~5,7~8', antenna='!ea06,ea17,ea20,ea26', datacolumn='data', keepflags=False, timebin='10s')''' | ||
field: Several fields have been removed which we won't be utilizing. We are only keeping fields 3-5. | field='3~5': Several fields have been removed which we won't be utilizing. We are only keeping fields 3-5.<br /> | ||
spw: Several spectral windows have been removed which were polluted with RFI, which neither auto-flagging nor hand flagging could remedy. We are keeping spectral windows 4,5,7,8. | spw='4~5,7~8': Several spectral windows have been removed which were polluted with RFI, which neither auto-flagging nor hand flagging could remedy. | ||
antenna: Several antennas have been removed (!), which at the time of the observation, didn't have an L-Band receiver installed. | We are keeping spectral windows 4,5,7,8.<br /> | ||
Time-averaged to 10-seconds, to reduce size and processing time when running tasks. | antenna='!ea06,ea17,ea20,ea26': Several antennas have been removed (!), which at the time of the observation, didn't have an L-Band receiver installed.<br /> | ||
timebin='10s': Time-averaged to 10-seconds, to reduce size and processing time when running tasks. | |||
== Unpack the Data == | == Unpack the Data == | ||
Line 48: | Line 52: | ||
Start CASA by typing '''casa''' on a terminal command line. If you have not used CASA before, some helpful tips are available on the [[Getting Started in CASA]] page. | Start CASA by typing '''casa''' on a terminal command line. If you have not used CASA before, some helpful tips are available on the [[Getting Started in CASA]] page. | ||
This guide has been written for CASA version 4.5. | This guide has been written for CASA version 4.5.2. Please confirm your version before proceeding by checking the message in the command line interface window or the CASA logger after startup. | ||
For this tutorial, we will be running tasks using the ''task (parameter = value)'' syntax. When called in this manner, all parameters not explicitly set will use their default values. | For this tutorial, we will be running tasks using the ''task (parameter = value)'' syntax. When called in this manner, all parameters not explicitly set will use their default values. | ||
Line 357: | Line 361: | ||
Strong RFI sources can give rise to the Gibbs phenomenon. This is seen by 'ringing', a zig-zag pattern across the channels that neighbor the strong, usually narrow RFI. To remedy this ringing across the frequency channels, we can employ the Hanning smoothing algorithm via the 'hanningsmooth2' task (an implementation based on {{mstransform}}. Hanning smoothing applies a triangle kernel across the pattern which reduces the ringing and thus reduces the number of channels that are affected by RFI. This smoothing procedure, however, will also decrease the spectral resolution by a factor of two. | Strong RFI sources can give rise to the Gibbs phenomenon. This is seen by 'ringing', a zig-zag pattern across the channels that neighbor the strong, usually narrow RFI. To remedy this ringing across the frequency channels, we can employ the Hanning smoothing algorithm via the 'hanningsmooth2' task (an implementation based on {{mstransform}}. Hanning smoothing applies a triangle kernel across the pattern which reduces the ringing and thus reduces the number of channels that are affected by RFI. This smoothing procedure, however, will also decrease the spectral resolution by a factor of two. | ||
The task allows for the creation of a new MS, or to directly operate on the requested column. For this tutorial, we will be directly operating on the data column and creating a new MS. Note that hanning-smoothing will remove amplitude spikes, it is therefore not recommended for spectral analysis related science, such as HI. Also note that hanning smoothing cannot be reversed once you apply it to your data. | |||
Let's create before and after images with the {{plotms}} task, to see the effects of hanning-smoothing the data. | Let's create before and after images with the {{plotms}} task, to see the effects of hanning-smoothing the data. | ||
Line 425: | Line 429: | ||
[[Image:Tfcrop.png|250px|thumb|right|TFcrop flagging results. Top row before, bottom row, after tfcrop.]] | [[Image:Tfcrop.png|250px|thumb|right|TFcrop flagging results. Top row before, bottom row, after tfcrop.]] | ||
As we iterate through the different scans and baselines, we can see the flagging we can apply, represented by the blue areas. Let's now apply these flags by changing the action parameter. | As we iterate through the different scans and baselines, we can see the flagging we can apply, represented by the blue areas. Click on the "Quit" button on the bottom right corner. Let's now apply these flags by changing the action parameter. | ||
<source lang="python"> | <source lang="python"> | ||
Line 446: | Line 450: | ||
</source> | </source> | ||
After tfcrop has gone through and flagged some of the worst RFI, we can inspect the log report and take note of how much has been flagged. | Iterate through several scans and baselines, and once you're ready to apply the flags, click on "Stop Display". After tfcrop has gone through and flagged some of the worst RFI, we can inspect the log report and take note of how much has been flagged. | ||
We can also use {{plotms}} to review the effects of using tfcrop and compare the image to the one created before applying tfcrop. We can see great improvements, especially for spectral window 0 and 2, which had some of the worst RFI. | We can also use {{plotms}} to review the effects of using tfcrop and compare the image to the one created before applying tfcrop. We can see great improvements, especially for spectral window 0 and 2, which had some of the worst RFI. | ||
Line 474: | Line 478: | ||
</source> | </source> | ||
The results indicate we have flagged a little over | The results indicate we have flagged a little over 26% of G55.7+3.4. We can now move on to the other auto-flagging algorithm, rflag. | ||
=== RFlag === | === RFlag === | ||
Line 678: | Line 682: | ||
</source> | </source> | ||
It appears that there are a | It appears that there are a several baselines which have consistently higher-amplitude than the others, indicating that they're probably contaminated by RFI. | ||
Use the plotms tools to identify | Use the plotms tools to identify the baselines (see above how to do that). We will flag just a few, ea04&ea16 and ea04&ea21. | ||
<source lang="python"> | <source lang="python"> | ||
# In CASA | # In CASA | ||
flagdata(vis='SNR_G55_10s-hanning.ms', antenna='ea04& | flagdata(vis='SNR_G55_10s-hanning.ms', antenna='ea04&ea16', spw='1', flagbackup=False) | ||
flagdata(vis='SNR_G55_10s-hanning.ms', antenna='ea04&ea21', spw='1', flagbackup=False) | flagdata(vis='SNR_G55_10s-hanning.ms', antenna='ea04&ea21', spw='1', flagbackup=False) | ||
</source> | </source> | ||
We will now run flagdata in summary mode to inspect how much data we have flagged | == Interactive Flagging == | ||
After plotting our data, it's obvious that there is still some amount of RFI present. We can use plotms to interactively flag points which look bad. A tutorial on flagging data interactively through plotms can be found [https://casaguides.nrao.edu/index.php?title=Data_flagging_with_plotms here]. Please note that flagging data through plotms will not create a backup, so it's important to use the flagmanager before deciding to mark your regions for flagging purposes. | |||
[[Image:corr.amp_v_freq.interactive_flagging.png|250px|thumb|right|Highlighting and interactively flagging RFI for scan 75.]] | |||
<source lang="python"> | |||
# In CASA | |||
flagmanager(vis='SNR_G55_10s-hanning.ms', mode='save', | |||
versionname='before_interactive_flagging') | |||
plotms(vis='SNR_G55_10s-hanning.ms', scan='30,75,120,165,190,235,303', xaxis='frequency', yaxis='amp', | |||
ydatacolumn='corrected', iteraxis='scan', correlation='RR,LL', coloraxis = 'spw') | |||
</source> | |||
Let's iterate through scans and view scan 75. We can see that spectral window 1 has a large amplitude spike. We can use the "mark regions" button (square with green plus sign) to highlight a majority of spike, and the "locate" button (magnifying glass) to give us information on the highlighted region. We will now click on the "flag" button (red flag) and we should see the plot update, having removed the highlighted region. Feel free to flag more data if you'd like. | |||
== Flagging Summary & Report == | |||
We will now run flagdata in summary mode to inspect how much data we have flagged in total. We can also create a plot of the percentage of flagged data with respect to frequency by setting display='report'. This parameter will also create a plot of the antennas, with the circle size representing the percentage of flagged data per antenna. | |||
<source lang="python"> | <source lang="python"> | ||
Line 706: | Line 729: | ||
</source> | </source> | ||
[[Image:Total_Flagged_plots.png|1200px|thumb|center|Total percentage of flagged data per spectral window (left). Percentage of data flagged from each antenna (right). A larger circle represents more flagged data for that antenna. ]] | |||
[[Image: | |||
So, as a result of the flagging, we have sacrificed 36% of the data for G55.7+3.4, and as expected, spectral windows 0 and 2 have the most flagged data. | |||
[[Main Page | ↵ '''CASAguides''']] | [[Main Page | ↵ '''CASAguides''']] | ||
Line 729: | Line 737: | ||
-- original: Miriam Hartman <br /> | -- original: Miriam Hartman <br /> | ||
--modifications: Lorant Sjouwerman (4.4.0, 2015/07/07) <br /> | --modifications: Lorant Sjouwerman (4.4.0, 2015/07/07) <br /> | ||
--modifications: Juergen Ott (4.5.2, 2016/04/13) <br /> | --modifications: Juergen Ott (4.5.2, 2016/04/13) <br /> | ||
--modifications: Jose Salcido (4.5.2, 2016/04/14) <br /> | |||
{{Checked 4.5.2}} | {{Checked 4.5.2}} |
Revision as of 15:44, 14 April 2016
- This CASA guide was designed for CASA 4.5.2
Overview
This CASA guide will cover a priori data flagging, including online flagging, shadowing, zero clipping, and quacking. It will also cover auto-flagging RFI (Radio Frequency Interference) via TFcrop (Time-Frequency crop) and rflag.
We will be utilizing data taken with the Karl G. Jansky, Very Large Array, of a supernova remnant G055.7+3.4.. The data were taken on August 23, 2010, in the first D-configuration for which the new wide-band capabilities of the WIDAR (Wideband Interferometric Digital ARchitecture) correlator were available. The 8-hour-long observation includes all available 1 GHz of bandwidth in L-band, from 1-2 GHz in frequency. The observations were made in 8-bit mode, which has the best dynamic range and is thus best suited for RFI excision.
The guide will often reference the CASA cookbook which is available online and can be downloaded as a pdf.
Obtaining the Data
A copy of the data (5.2GB) can be downloaded from http://casa.nrao.edu/Data/EVLA/SNRG55/SNR_G55_10s.tar.gz These data are already in CASA MeasurementSet (MS) format and were prepared specifically for this guide.
In the following we explain how the data were processed as it has implications for later flagging steps. First, the data were loaded from the NRAO archive. On the archive search form, specify "Archive File ID" to "AB1345_sb1800808_1.55431.004049953706" and select "SDM-BDF dataset (all files)" as the data download option on the following page. But be aware that the full dataset has 170GB!
Once the SDM is downloaded, create a MeasurementSet with either task importevla or importasdm. Here we are using the second option:
importasdm(asdm='AB1345_sb1800808_1.55431.004049953706', vis='SNR_G55.ms', process_flags=True, tbuff=1.5, applyflags=False, outfile='SNR_G55.ms.onlineflags.txt', flagbackup=False)
process_flags=True: This parameter controls the creation of online flags from the Flag.xml SDM table. It will create online flags in the FLAG_CMD sub-table within the MS (more on this later).
tbuff=1.5: This parameter adds a time "buffer" padding to the flags in both directions to deal with timing mismatches. This is important for JVLA data taken before April 2011. This value should be set to 1.5x integration time. This particular observation had 1 second integrations. (CASA Cookbook section 2.2.2 and 3.5.1.3)
applyflags=False: We will apply these flags later in the tutorial.
outfile='SNR_G55.ms.onlineflags.txt': This will create a text file with a list of online flags that can be applied. Created just for convenience, and won't be used during the tutorial.
Split and time-averaged using the split task. For CASA 4.5.3 we use the split2() implementation:
split2(vis='SNR_G55.ms', outputvis='SNR_G55_10s.ms', field='3~5', spw='4~5,7~8', antenna='!ea06,ea17,ea20,ea26', datacolumn='data', keepflags=False, timebin='10s')
field='3~5': Several fields have been removed which we won't be utilizing. We are only keeping fields 3-5.
spw='4~5,7~8': Several spectral windows have been removed which were polluted with RFI, which neither auto-flagging nor hand flagging could remedy. We are keeping spectral windows 4,5,7,8.
antenna='!ea06,ea17,ea20,ea26': Several antennas have been removed (!), which at the time of the observation, didn't have an L-Band receiver installed.
timebin='10s': Time-averaged to 10-seconds, to reduce size and processing time when running tasks.
Unpack the Data
Once you've downloaded the file from the link above, let's untar it:
tar -xzvf SNR_G55_10s.tar.gz
That will create a directory called SNR_G55_10s.ms (6.1GB), which is the MS.
Starting CASA
Start CASA by typing casa on a terminal command line. If you have not used CASA before, some helpful tips are available on the Getting Started in CASA page.
This guide has been written for CASA version 4.5.2. Please confirm your version before proceeding by checking the message in the command line interface window or the CASA logger after startup.
For this tutorial, we will be running tasks using the task (parameter = value) syntax. When called in this manner, all parameters not explicitly set will use their default values.
Preliminary Data Evaluation
As a first step, use listobs to have a look at the MS:
# In CASA
listobs(vis='SNR_G55_10s.ms', listfile='SNR_G55_10s.listobs')
- listfile: Creates a text document "SNR_G55_10s.listobs" with details for the observation:
================================================================================ MeasurementSet Name: /lustre/aoc/sciops/jott/casa/topicalguide/SNR_G55_10s.ms MS Version 2 ================================================================================ Observer: Dr. Sanjay Sanjay Bhatnagar Project: uid://evla/pdb/1072564 Observation: EVLA Data records: 3024000 Total elapsed time = 26926 seconds Observed from 23-Aug-2010/00:56:36.0 to 23-Aug-2010/08:25:22.0 (UTC) ObservationID = 0 ArrayID = 0 Date Timerange (UTC) Scan FldId FieldName nRows SpwIds Average Interval(s) ScanIntent 23-Aug-2010/00:56:36.0 - 00:58:06.0 14 0 J1925+2106 10080 [0,1,2,3] [10, 10, 10, 10] [CALIBRATE_PHASE#UNSPECIFIED] 00:58:06.0 - 00:59:36.0 15 0 J1925+2106 10080 [0,1,2,3] [10, 10, 10, 10] [CALIBRATE_PHASE#UNSPECIFIED] 00:59:36.0 - 01:01:05.0 16 0 J1925+2106 10080 [0,1,2,3] [9.89, 9.89, 9.89, 9.89] [CALIBRATE_PHASE#UNSPECIFIED] 01:01:05.0 - 01:02:35.0 17 0 J1925+2106 10080 [0,1,2,3] [10, 10, 10, 10] [CALIBRATE_PHASE#UNSPECIFIED] 01:02:35.0 - 01:04:05.0 18 0 J1925+2106 10080 [0,1,2,3] [10, 10, 10, 10] [CALIBRATE_PHASE#UNSPECIFIED] 01:04:05.0 - 01:05:34.0 19 0 J1925+2106 10080 [0,1,2,3] [9.89, 9.89, 9.89, 9.89] [CALIBRATE_PHASE#UNSPECIFIED] 01:05:34.0 - 01:07:04.0 20 0 J1925+2106 10080 [0,1,2,3] [10, 10, 10, 10] [CALIBRATE_PHASE#UNSPECIFIED] 01:07:04.0 - 01:08:34.0 21 1 G55.7+3.4 10080 [0,1,2,3] [10, 10, 10, 10] [OBSERVE_TARGET#UNSPECIFIED] 01:08:34.0 - 01:10:04.0 22 1 G55.7+3.4 10080 [0,1,2,3] [10, 10, 10, 10] [OBSERVE_TARGET#UNSPECIFIED] 01:10:04.0 - 01:11:34.0 23 1 G55.7+3.4 10080 [0,1,2,3] [10, 10, 10, 10] [OBSERVE_TARGET#UNSPECIFIED] 01:11:34.0 - 01:13:03.0 24 1 G55.7+3.4 10080 [0,1,2,3] [9.89, 9.89, 9.89, 9.89] [OBSERVE_TARGET#UNSPECIFIED] 01:13:03.0 - 01:14:33.0 25 1 G55.7+3.4 10080 [0,1,2,3] [10, 10, 10, 10] [OBSERVE_TARGET#UNSPECIFIED] 01:14:33.0 - 01:16:03.0 26 1 G55.7+3.4 10080 [0,1,2,3] [10, 10, 10, 10] [OBSERVE_TARGET#UNSPECIFIED] 01:16:03.0 - 01:17:33.0 27 1 G55.7+3.4 10080 [0,1,2,3] [10, 10, 10, 10] [OBSERVE_TARGET#UNSPECIFIED] 01:17:33.0 - 01:19:02.0 28 1 G55.7+3.4 10080 [0,1,2,3] [9.89, 9.89, 9.89, 9.89] [OBSERVE_TARGET#UNSPECIFIED] 01:19:02.0 - 01:20:32.0 29 1 G55.7+3.4 10080 [0,1,2,3] [10, 10, 10, 10] [OBSERVE_TARGET#UNSPECIFIED] 01:20:32.0 - 01:22:02.0 30 1 G55.7+3.4 10080 [0,1,2,3] [10, 10, 10, 10] [OBSERVE_TARGET#UNSPECIFIED] 01:22:02.0 - 01:23:32.0 31 1 G55.7+3.4 10080 [0,1,2,3] [10, 10, 10, 10] [OBSERVE_TARGET#UNSPECIFIED] 01:23:32.0 - 01:25:01.0 32 1 G55.7+3.4 10080 [0,1,2,3] [9.89, 9.89, 9.89, 9.89] [OBSERVE_TARGET#UNSPECIFIED] 01:25:01.0 - 01:26:31.0 33 1 G55.7+3.4 10080 [0,1,2,3] [10, 10, 10, 10] [OBSERVE_TARGET#UNSPECIFIED] 01:26:31.0 - 01:28:01.0 34 1 G55.7+3.4 10080 [0,1,2,3] [10, 10, 10, 10] [OBSERVE_TARGET#UNSPECIFIED] 01:28:01.0 - 01:29:31.0 35 1 G55.7+3.4 10080 [0,1,2,3] [10, 10, 10, 10] [OBSERVE_TARGET#UNSPECIFIED] 01:29:31.0 - 01:31:00.0 36 1 G55.7+3.4 10080 [0,1,2,3] [9.89, 9.89, 9.89, 9.89] [OBSERVE_TARGET#UNSPECIFIED] 01:31:00.0 - 01:32:30.0 37 1 G55.7+3.4 10080 [0,1,2,3] [10, 10, 10, 10] [OBSERVE_TARGET#UNSPECIFIED] 01:32:30.0 - 01:34:00.0 38 1 G55.7+3.4 10080 [0,1,2,3] [10, 10, 10, 10] [OBSERVE_TARGET#UNSPECIFIED] 01:34:00.0 - 01:35:30.0 39 1 G55.7+3.4 10080 [0,1,2,3] [10, 10, 10, 10] [OBSERVE_TARGET#UNSPECIFIED] 01:35:30.0 - 01:36:59.0 40 1 G55.7+3.4 10080 [0,1,2,3] [9.89, 9.89, 9.89, 9.89] [OBSERVE_TARGET#UNSPECIFIED] 01:36:59.0 - 01:38:29.0 41 0 J1925+2106 10080 [0,1,2,3] [10, 10, 10, 10] [CALIBRATE_PHASE#UNSPECIFIED] 01:38:29.0 - 01:39:59.0 42 0 J1925+2106 10080 [0,1,2,3] [10, 10, 10, 10] [CALIBRATE_PHASE#UNSPECIFIED] 01:39:59.0 - 01:41:29.0 43 0 J1925+2106 10080 [0,1,2,3] [10, 10, 10, 10] [CALIBRATE_PHASE#UNSPECIFIED] 01:41:29.0 - 01:42:58.0 44 1 G55.7+3.4 10080 [0,1,2,3] [9.89, 9.89, 9.89, 9.89] [OBSERVE_TARGET#UNSPECIFIED] <snip> 08:11:54.0 - 08:13:24.0 305 1 G55.7+3.4 10080 [0,1,2,3] [10, 10, 10, 10] [OBSERVE_TARGET#UNSPECIFIED] 08:13:24.0 - 08:14:54.0 306 1 G55.7+3.4 10080 [0,1,2,3] [10, 10, 10, 10] [OBSERVE_TARGET#UNSPECIFIED] 08:14:54.0 - 08:16:24.0 307 2 0542+498=3C147 10080 [0,1,2,3] [10, 10, 10, 10] [CALIBRATE_AMPLI#UNSPECIFIED,CALIBRATE_BANDPASS#UNSPECIFIED,UNSPECIFIED#UNSPECIFIED] 08:16:24.0 - 08:17:54.0 308 2 0542+498=3C147 10080 [0,1,2,3] [10, 10, 10, 10] [CALIBRATE_AMPLI#UNSPECIFIED,CALIBRATE_BANDPASS#UNSPECIFIED,UNSPECIFIED#UNSPECIFIED] 08:17:54.0 - 08:19:23.0 309 2 0542+498=3C147 10080 [0,1,2,3] [9.89, 9.89, 9.89, 9.89] [CALIBRATE_AMPLI#UNSPECIFIED,CALIBRATE_BANDPASS#UNSPECIFIED,UNSPECIFIED#UNSPECIFIED] 08:19:23.0 - 08:20:53.0 310 2 0542+498=3C147 10080 [0,1,2,3] [10, 10, 10, 10] [CALIBRATE_AMPLI#UNSPECIFIED,CALIBRATE_BANDPASS#UNSPECIFIED,UNSPECIFIED#UNSPECIFIED] 08:20:53.0 - 08:22:23.0 311 2 0542+498=3C147 10080 [0,1,2,3] [10, 10, 10, 10] [CALIBRATE_AMPLI#UNSPECIFIED,CALIBRATE_BANDPASS#UNSPECIFIED,UNSPECIFIED#UNSPECIFIED] 08:22:23.0 - 08:23:52.0 312 2 0542+498=3C147 10080 [0,1,2,3] [9.89, 9.89, 9.89, 9.89] [CALIBRATE_AMPLI#UNSPECIFIED,CALIBRATE_BANDPASS#UNSPECIFIED,UNSPECIFIED#UNSPECIFIED] 08:23:52.0 - 08:25:22.0 313 2 0542+498=3C147 10080 [0, 1, 2, 3] [10, 10, 10, 10] [CALIBRATE_AMPLI#UNSPECIFIED,CALIBRATE_BANDPASS#UNSPECIFIED,UNSPECIFIED#UNSPECIFIED] (nRows = Total number of rows per scan) Fields: 3 ID Code Name RA Decl Epoch SrcId nRows 0 D J1925+2106 19:25:59.605371 +21.06.26.16218 J2000 0 433440 1 NONE G55.7+3.4 19:21:40.000000 +21.45.00.00000 J2000 1 2520000 2 N 0542+498=3C147 05:42:36.137916 +49.51.07.23356 J2000 2 70560 Spectral Windows: (4 unique spectral windows and 1 unique polarization setups) SpwID Name #Chans Frame Ch0(MHz) ChanWid(kHz) TotBW(kHz) CtrFreq(MHz) BBC Num Corrs 0 Subband:0 64 TOPO 1256.000 2000.000 128000.0 1319.0000 4 RR RL LR LL 1 Subband:2 64 TOPO 1384.000 2000.000 128000.0 1447.0000 4 RR RL LR LL 2 Subband:1 64 TOPO 1648.000 2000.000 128000.0 1711.0000 8 RR RL LR LL 3 Subband:0 64 TOPO 1776.000 2000.000 128000.0 1839.0000 8 RR RL LR LL Sources: 12 ID Name SpwId RestFreq(MHz) SysVel(km/s) 0 J1925+2106 0 - - 0 J1925+2106 1 - - 0 J1925+2106 2 - - 0 J1925+2106 3 - - 1 G55.7+3.4 0 - - 1 G55.7+3.4 1 - - 1 G55.7+3.4 2 - - 1 G55.7+3.4 3 - - 2 0542+498=3C147 0 - - 2 0542+498=3C147 1 - - 2 0542+498=3C147 2 - - 2 0542+498=3C147 3 - - Antennas: 27: ID Name Station Diam. Long. Lat. Offset from array center (m) ITRF Geocentric coordinates (m) East North Elevation x y z 0 ea01 W09 25.0 m -107.37.25.2 +33.53.51.0 -521.9416 -332.7766 -1.2001 -1601710.017000 -5042006.925200 3554602.355600 1 ea02 E02 25.0 m -107.37.04.4 +33.54.01.1 9.8240 -20.4293 -2.7806 -1601150.060300 -5042000.619800 3554860.729400 2 ea03 E09 25.0 m -107.36.45.1 +33.53.53.6 506.0564 -251.8670 -3.5825 -1600715.950800 -5042273.187000 3554668.184500 3 ea04 W01 25.0 m -107.37.05.9 +33.54.00.5 -27.3562 -41.3030 -2.7418 -1601189.030140 -5042000.493300 3554843.425700 4 ea05 W08 25.0 m -107.37.21.6 +33.53.53.0 -432.1167 -272.1478 -1.5054 -1601614.091000 -5042001.652900 3554652.509300 5 ea06 N06 25.0 m -107.37.06.9 +33.54.10.3 -54.0649 263.8778 -4.2273 -1601162.591000 -5041828.999000 3555095.896400 6 ea07 E05 25.0 m -107.36.58.4 +33.53.58.8 164.9788 -92.8032 -2.5268 -1601014.462000 -5042086.252000 3554800.799800 7 ea08 N01 25.0 m -107.37.06.0 +33.54.01.8 -30.8810 -1.4664 -2.8597 -1601185.634945 -5041978.156586 3554876.424700 8 ea09 E06 25.0 m -107.36.55.6 +33.53.57.7 236.9058 -126.3369 -2.4443 -1600951.588000 -5042125.911000 3554773.012300 9 ea10 N03 25.0 m -107.37.06.3 +33.54.04.8 -39.0773 93.0192 -3.3330 -1601177.376760 -5041925.073200 3554954.584100 10 ea11 E04 25.0 m -107.37.00.8 +33.53.59.7 102.8054 -63.7682 -2.6414 -1601068.790300 -5042051.910200 3554824.835300 11 ea12 E08 25.0 m -107.36.48.9 +33.53.55.1 407.8285 -206.0065 -3.2272 -1600801.926000 -5042219.366500 3554706.448200 12 ea13 N07 25.0 m -107.37.07.2 +33.54.12.9 -61.1037 344.2331 -4.6138 -1601155.635800 -5041783.843800 3555162.374100 13 ea15 W06 25.0 m -107.37.15.6 +33.53.56.4 -275.8288 -166.7451 -2.0590 -1601447.198000 -5041992.502500 3554739.687600 14 ea16 W02 25.0 m -107.37.07.5 +33.54.00.9 -67.9687 -26.5614 -2.7175 -1601225.255200 -5041980.383590 3554855.675000 15 ea17 W07 25.0 m -107.37.18.4 +33.53.54.8 -349.9877 -216.7509 -1.7975 -1601526.387300 -5041996.840100 3554698.327400 16 ea18 N09 25.0 m -107.37.07.8 +33.54.19.0 -77.4346 530.6273 -5.5859 -1601139.485100 -5041679.036800 3555316.533200 17 ea19 W04 25.0 m -107.37.10.8 +33.53.59.1 -152.8599 -83.8054 -2.4614 -1601315.893000 -5041985.320170 3554808.304600 18 ea20 N05 25.0 m -107.37.06.7 +33.54.08.0 -47.8454 192.6015 -3.8723 -1601168.786100 -5041869.054000 3555036.936000 19 ea21 E01 25.0 m -107.37.05.7 +33.53.59.2 -23.8638 -81.1510 -2.5851 -1601192.467800 -5042022.856800 3554810.438800 20 ea22 N04 25.0 m -107.37.06.5 +33.54.06.1 -42.6239 132.8436 -3.5494 -1601173.979400 -5041902.657700 3554987.517500 21 ea23 E07 25.0 m -107.36.52.4 +33.53.56.5 318.0509 -164.1850 -2.6957 -1600880.571400 -5042170.388000 3554741.457400 22 ea24 W05 25.0 m -107.37.13.0 +33.53.57.8 -210.0959 -122.3887 -2.2577 -1601377.009500 -5041988.665500 3554776.393400 23 ea25 N02 25.0 m -107.37.06.2 +33.54.03.5 -35.6245 53.1806 -3.1345 -1601180.861480 -5041947.453400 3554921.628700 24 ea26 W03 25.0 m -107.37.08.9 +33.54.00.1 -105.3447 -51.7177 -2.6037 -1601265.153600 -5041982.533050 3554834.858400 25 ea27 E03 25.0 m -107.37.02.8 +33.54.00.5 50.6641 -39.4835 -2.7273 -1601114.365500 -5042023.151800 3554844.944000 26 ea28 N08 25.0 m -107.37.07.5 +33.54.15.8 -68.9057 433.1889 -5.0602 -1601147.940400 -5041733.837000 3555235.956000
- J1925+2106, field ID 0: Phase Calibrator;
- G55.7+3.4, field ID 1: The Supernova Remnant;
- 0542+498=3C147, field ID 2: Amplitude/Bandpass Calibrator
We can also see that these sources have associated "scan intents", which indicate their function in the observation. Note that you can select sources based on their intents in certain CASA tasks. The various scan intents in this data set are:
- CALIBRATE_PHASE indicates that this is a scan to be used for gain calibration;
- OBSERVE_TARGET indicates that this is the science target;
- CALIBRATE_AMPLI indicates that this is to be used for flux calibration; and
- CALIBRATE_BANDPASS indicates that these scans are to be used for bandpass calibration.
Note that 3C147 is to be used for both flux and bandpass calibration.
It's important to also note that the antennas have a name and ID associated with them. For example antenna ID 15 is named ea17 ( The "ea" stemming from the Expanded VLA project). When specifying an antenna within a task parameter, we will mainly reference them by name.
We can see the antenna configuration for this observation by using plotants:
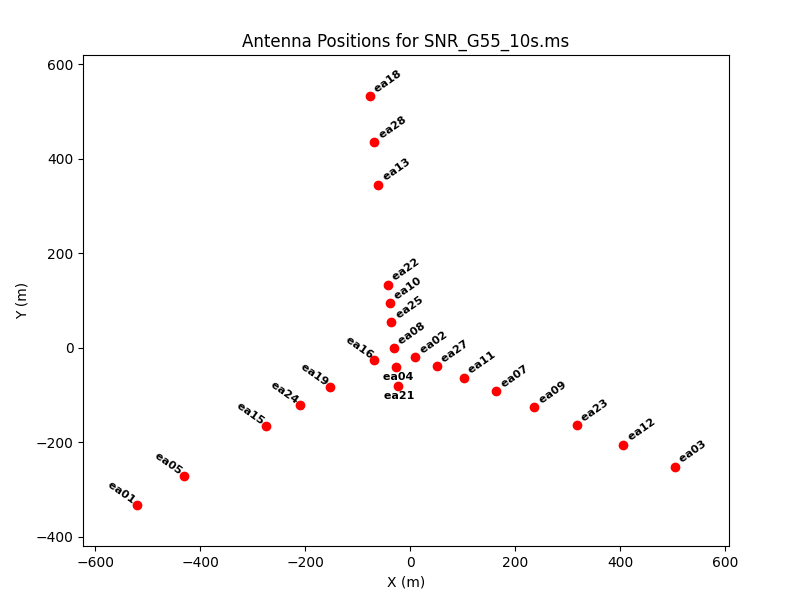
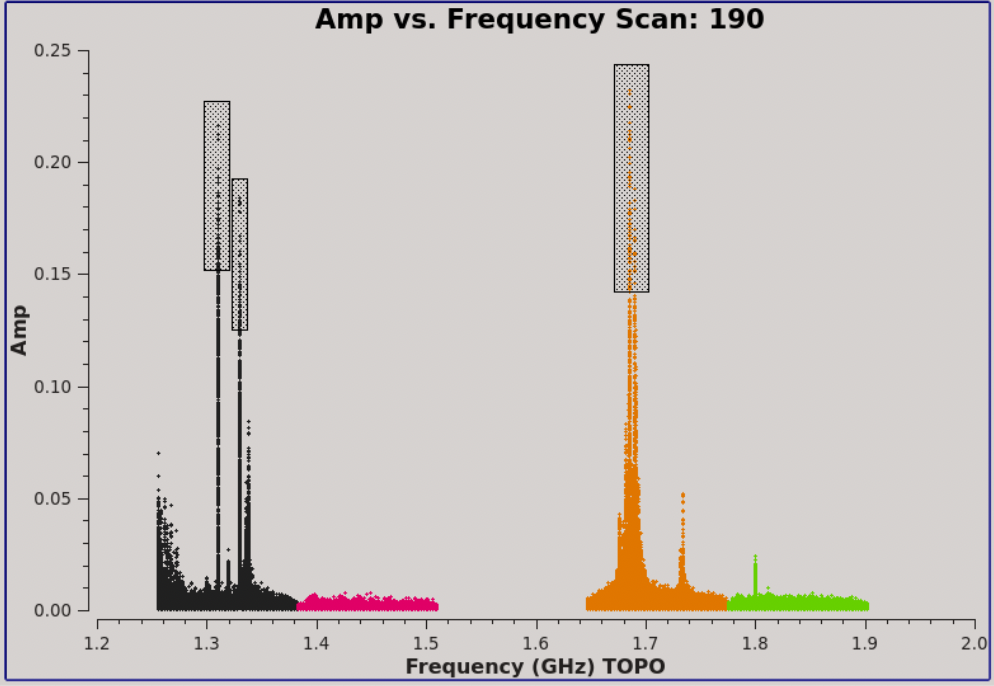
# In CASA
plotants(vis='SNR_G55_10s.ms', figfile='SNR_G55_10s.plotants.png')
This shows that antennas ea01, ea03, and ea18 were on the extreme ends of the west, east, and north arms, respectively. The antenna position diagram is particularly useful as a guide to help determine which antenna to use as the reference antenna later during calibration. Note that antennas on stations 8 of each arm (N08, E08, W08) do not get moved during array reconfigurations, they can therefore at times be good choices as reference antennas. In this case, we'll probably want to choose something closer to the center of the array, with no shadowing.
We may also inspect the raw data using plotms. To start with, lets look at a subset of scans on the supernova remnant:
# In CASA
plotms(vis='SNR_G55_10s.ms', scan='30,75,120,165,190,235,303', antenna='ea24', xaxis='freq',
yaxis='amp', coloraxis='spw', iteraxis='scan', correlation='RR,LL')
- coloraxis='spw': Parameter indicates that a different color will be assigned to each spectral window.
- antenna='ea24' : We chose only information for antenna ea24.
- iteraxis='scan': Parameter tells plotms to display a new plot for each scan.
- correlation='RR,LL': We just want to display the right and left circular polarizations, without the cross-hand terms.
Flipping through to scan 190, we can see that there is significant time and frequency variable RFI present in the observation, as seen by the large spikes in amplitude. In particular, we can see that several spectral windows are quite badly affected. To determine which spectral windows they are, click on the "Mark Regions" tool at the bottom of the plotms GUI (the open box with a green "plus" sign). Use the mouse to select a few of the highest-amplitude points in each of the spectral windows. Click on the "Locate" button (magnifying glass). Information about the selected areas should now display in the logger window. For example:
Frequency in [1.30662 1.31407] or [1.32377 1.33569] or [1.67866 1.69581], Amp in [0.16871 0.217097] or [0.153226 0.186613] or [0.172581 0.235968]: Scan=190 Field=G55.7+3.4[1] Time=2010/08/23/05:19:58.0 BL=ea16@W02 & ea24@W05[14&22] Spw=0 Chan=27 Freq=1.31 Corr=RR X=1.31 Y=0.17187 (1718/144/1718) Scan=190 Field=G55.7+3.4[1] Time=2010/08/23/05:19:58.0 BL=ea19@W04 & ea24@W05[17&22] Spw=0 Chan=27 Freq=1.31 Corr=LL X=1.31 Y=0.178607 (1975/144/1975) Scan=190 Field=G55.7+3.4[1] Time=2010/08/23/05:19:58.0 BL=ea22@N04 & ea24@W05[20&22] Spw=0 Chan=37 Freq=1.33 Corr=RR X=1.33 Y=0.18105 (2250/144/2250) Scan=190 Field=G55.7+3.4[1] Time=2010/08/23/05:20:08.0 BL=ea19@W04 & ea24@W05[17&22] Spw=0 Chan=27 Freq=1.31 Corr=LL X=1.31 Y=0.21228 (1975/145/1975) Scan=190 Field=G55.7+3.4[1] Time=2010/08/23/05:20:08.0 BL=ea21@E01 & ea24@W05[19&22] Spw=0 Chan=27 Freq=1.31 Corr=LL X=1.31 Y=0.181632 (2103/145/2103) Scan=190 Field=G55.7+3.4[1] Time=2010/08/23/05:20:08.0 BL=ea22@N04 & ea24@W05[20&22] Spw=0 Chan=37 Freq=1.33 Corr=RR X=1.33 Y=0.167068 (2250/145/2250) Scan=190 Field=G55.7+3.4[1] Time=2010/08/23/05:20:28.0 BL=ea19@W04 & ea24@W05[17&22] Spw=0 Chan=27 Freq=1.31 Corr=LL X=1.31 Y=0.216252 (1818323722/147/1975) Scan=190 Field=G55.7+3.4[1] Time=2010/08/23/05:20:28.0 BL=ea22@N04 & ea24@W05[20&22] Spw=0 Chan=37 Freq=1.33 Corr=RR X=1.33 Y=0.182341 (1818323997/147/2250) Scan=190 Field=G55.7+3.4[1] Time=2010/08/23/05:20:38.0 BL=ea07@E05 & ea24@W05[6&22] Spw=0 Chan=27 Freq=1.31 Corr=LL X=1.31 Y=0.175032 (1866691864/148/695) Scan=190 Field=G55.7+3.4[1] Time=2010/08/23/05:20:38.0 BL=ea09@E06 & ea24@W05[8&22] Spw=0 Chan=27 Freq=1.31 Corr=RR X=1.31 Y=0.176728 (1866692119/148/950) Scan=190 Field=G55.7+3.4[1] Time=2010/08/23/05:20:38.0 BL=ea16@W02 & ea24@W05[14&22] Spw=0 Chan=27 Freq=1.31 Corr=LL X=1.31 Y=0.170435 (1866692888/148/1719) Scan=190 Field=G55.7+3.4[1] Time=2010/08/23/05:20:38.0 BL=ea19@W04 & ea24@W05[17&22] Spw=0 Chan=27 Freq=1.31 Corr=LL X=1.31 Y=0.210123 (1866693144/148/1975) Scan=190 Field=G55.7+3.4[1] Time=2010/08/23/05:20:38.0 BL=ea21@E01 & ea24@W05[19&22] Spw=0 Chan=27 Freq=1.31 Corr=LL X=1.31 Y=0.185065 (1866693272/148/2103) Scan=190 Field=G55.7+3.4[1] Time=2010/08/23/05:20:38.0 BL=ea22@N04 & ea24@W05[20&22] Spw=0 Chan=37 Freq=1.33 Corr=RR X=1.33 Y=0.158359 (1866693419/148/2250) Scan=190 Field=G55.7+3.4[1] Time=2010/08/23/05:20:38.0 BL=ea24@W05 & ea27@E03[22&25] Spw=0 Chan=27 Freq=1.31 Corr=RR X=1.31 Y=0.190659 (1866693783/148/2614) Scan=190 Field=G55.7+3.4[1] Time=2010/08/23/05:20:48.0 BL=ea21@E01 & ea24@W05[19&22] Spw=0 Chan=27 Freq=1.31 Corr=LL X=1.31 Y=0.197052 (1852669347/149/2103) Scan=190 Field=G55.7+3.4[1] Time=2010/08/23/05:20:48.0 BL=ea22@N04 & ea24@W05[20&22] Spw=0 Chan=37 Freq=1.33 Corr=RR X=1.33 Y=0.183905 (1852669494/149/2250) Scan=190 Field=G55.7+3.4[1] Time=2010/08/23/05:20:48.0 BL=ea24@W05 & ea27@E03[22&25] Spw=0 Chan=27 Freq=1.31 Corr=RR X=1.31 Y=0.170542 (1852669858/149/2614) Scan=190 Field=G55.7+3.4[1] Time=2010/08/23/05:20:58.0 BL=ea21@E01 & ea24@W05[19&22] Spw=0 Chan=27 Freq=1.31 Corr=LL X=1.31 Y=0.179017 (1668509051/150/2103) Scan=190 Field=G55.7+3.4[1] Time=2010/08/23/05:20:58.0 BL=ea22@N04 & ea24@W05[20&22] Spw=0 Chan=37 Freq=1.33 Corr=RR X=1.33 Y=0.177841 (1668509198/150/2250) Scan=190 Field=G55.7+3.4[1] Time=2010/08/23/05:21:08.0 BL=ea21@E01 & ea24@W05[19&22] Spw=0 Chan=27 Freq=1.31 Corr=LL X=1.31 Y=0.193158 (1866681459/151/2103) Scan=190 Field=G55.7+3.4[1] Time=2010/08/23/05:19:58.0 BL=ea19@W04 & ea24@W05[17&22] Spw=2 Chan=19 Freq=1.686 Corr=RR X=1.686 Y=0.209811 (4844192/162/1958) Scan=190 Field=G55.7+3.4[1] Time=2010/08/23/05:19:58.0 BL=ea19@W04 & ea24@W05[17&22] Spw=2 Chan=19 Freq=1.686 Corr=LL X=1.686 Y=0.224424 (4844193/162/1959) Scan=190 Field=G55.7+3.4[1] Time=2010/08/23/05:20:08.0 BL=ea19@W04 & ea24@W05[17&22] Spw=2 Chan=19 Freq=1.686 Corr=RR X=1.686 Y=0.231918 (1374179750/163/1958) Scan=190 Field=G55.7+3.4[1] Time=2010/08/23/05:20:08.0 BL=ea19@W04 & ea24@W05[17&22] Spw=2 Chan=19 Freq=1.686 Corr=LL X=1.686 Y=0.209708 (1374179751/163/1959) Scan=190 Field=G55.7+3.4[1] Time=2010/08/23/05:20:18.0 BL=ea08@N01 & ea24@W05[7&22] Spw=2 Chan=19 Freq=1.686 Corr=RR X=1.686 Y=0.17744 (2898499/164/806) Scan=190 Field=G55.7+3.4[1] Time=2010/08/23/05:20:18.0 BL=ea10@N03 & ea24@W05[9&22] Spw=2 Chan=19 Freq=1.686 Corr=LL X=1.686 Y=0.177235 (2898756/164/1063) Scan=190 Field=G55.7+3.4[1] Time=2010/08/23/05:20:18.0 BL=ea19@W04 & ea24@W05[17&22] Spw=2 Chan=19 Freq=1.686 Corr=RR X=1.686 Y=0.230346 (2899651/164/1958) Scan=190 Field=G55.7+3.4[1] Time=2010/08/23/05:20:18.0 BL=ea19@W04 & ea24@W05[17&22] Spw=2 Chan=19 Freq=1.686 Corr=LL X=1.686 Y=0.199502 (2899652/164/1959) Scan=190 Field=G55.7+3.4[1] Time=2010/08/23/05:20:28.0 BL=ea08@N01 & ea24@W05[7&22] Spw=2 Chan=19 Freq=1.686 Corr=RR X=1.686 Y=0.177315 (6083110/165/806) Scan=190 Field=G55.7+3.4[1] Time=2010/08/23/05:20:28.0 BL=ea10@N03 & ea24@W05[9&22] Spw=2 Chan=19 Freq=1.686 Corr=LL X=1.686 Y=0.17881 (6083367/165/1063) Scan=190 Field=G55.7+3.4[1] Time=2010/08/23/05:20:28.0 BL=ea19@W04 & ea24@W05[17&22] Spw=2 Chan=19 Freq=1.686 Corr=RR X=1.686 Y=0.217573 (6084262/165/1958) Found 45 points (45 unflagged) among 101376 in 0s.
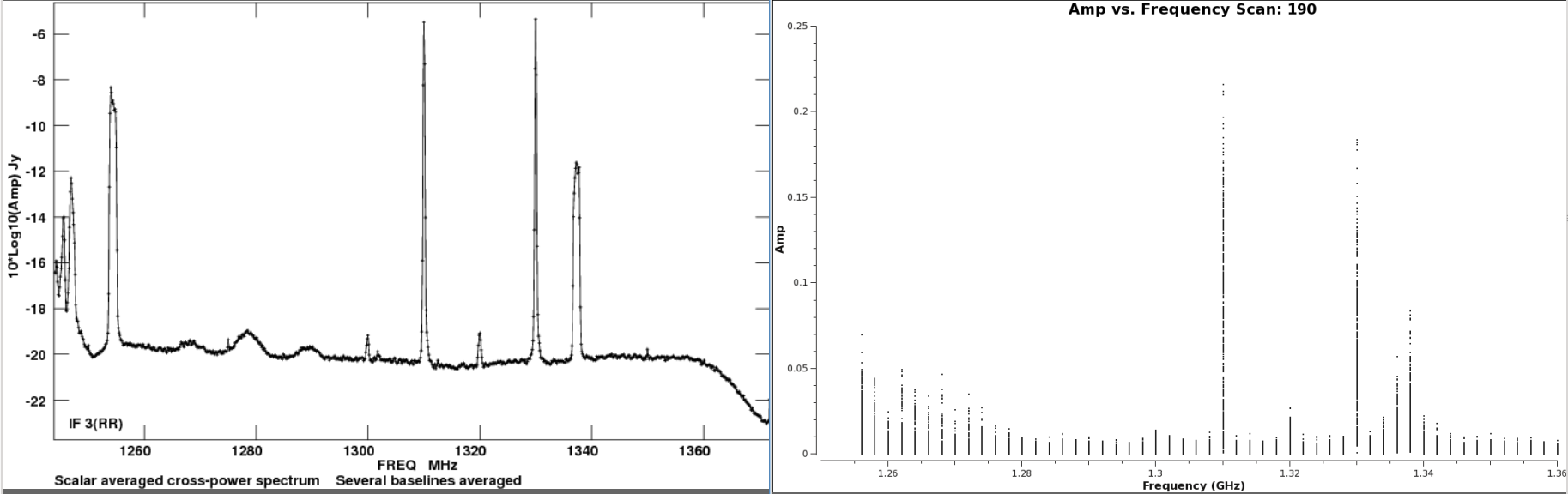
We can see that Spw 0 and 2, are the worst affected by RFI. Also, we get the corresponding frequency where the RFI is present, which appears to be 1.31 and 1.33GHz for spectral window 0, and 1.686GHz for spectral window 2.
The VLA has obtained RFI scans over its frequency range. And the results are presented on the Radio Frequency Interference website. Most of the RFI features can be identified, e.g. as radar, communications, satellites, airplanes, or birdies, i.e. RFI generated by the VLA electronics itself. For our observations, we can inspect the L-band specific RFI plots website. We find that the 1310 and 1330 MHz features are due to FAA ASR radars, and the one at 1686MHz is most likely due to a GOES weather satellite. We can compare the plots of the FAA ASR radar from the website, with that of spw 0, and see that the spikes are practically identical and fall within the same frequencies.
Identifying Problematic Antennas from the Operator Logs
We first check the operator log for the observation to see if there were any issues noted during the run that need to be addressed. The logs are available on this website and the observing log file for our observation can be found here.
The log has various pieces of information, including the start/end times for the observation, frequency bands used, weather, baseline information for recently moved antennas, and any outages or issues that may have been encountered. We can see that antenna ea07 may need position corrections (see one of our calibration tutorials, e.g. IRC+10216 on how to fix this), and several antennas are missing an L-Band receiver, including ea06, ea17, ea20, and ea26. These antennas were already removed from the dataset used here (as explained above in "Obtaining the Data") and we can continue with online flagging.
Online Flags
At the time of importing from the SDM-BDF raw data to a MS, we chose to process the online flags from the Flags.xml file to the FLAG_CMD sub-table within the MS. We also created a txt document which includes a list of online flags.
The Flags.xml file holds information of flags created during the observation, such as subreflector issues, and antennas not being on source. We will now want to apply these online flags to the data, but first, let's create a plot of the flags we are about to apply to get an idea of what will be flagged.
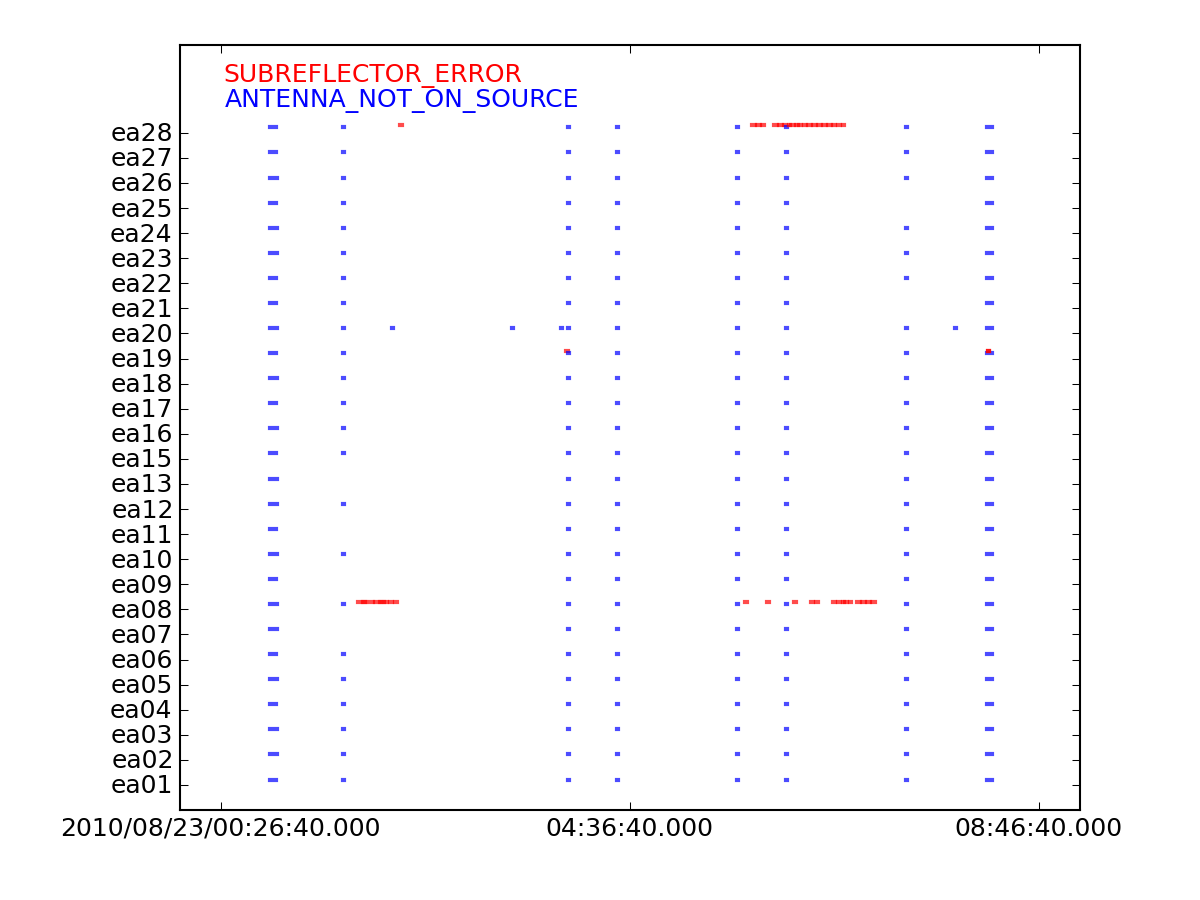
We will employ the flagcmd task to apply the online flags.
# In CASA
flagcmd(vis='SNR_G55_10s.ms', inpmode='table', reason='any', action='plot', plotfile='flaggingreason_vs_time.png')
We can see several instances of online flagging in the created image. Most notably, ea28 and ea08 had some subreflector issues througout the observation. Online flags are instances of possible missing data, including:
- ANTENNA_NOT_ON_SOURCE
The VLA antennas have slewing speeds of 20 degrees per minute in azimuth, and 40 degrees per minute in elevation. Some antennas are slower than others, and may take a few more seconds to reach the next source. The antennas can also take a few seconds to settle down due to small oscillations after having slewed.
- SUBREFLECTOR_ERROR
The FRM (Focus Rotation Mount) located at the apex of the antennas, is responsible for focusing the incoming radio signal to the corresponding receiver. They can at times have issues with their focus and/or rotation axes. Being off target, so much as a few fractions of a degree can result in loss of data, depending on the frequency being observed.
Now that we've plotted the online flags, we will apply them to the MS.
# In CASA
flagcmd(vis='SNR_G55_10s.ms', inpmode='table', reason='any', action='apply', flagbackup=False)
The CASA logger should report the progress as the task applies these flags in chunks. Once it has finished, it will report on the percentage of data that has been flagged.
Shadowed Antennas
Since this is the most compact VLA configuration, there may be instances where one antenna blocks, or "shadows" another. flagdata (CASA Cookbook 3.4) can determine shadowed antennas by their location and observation direction:
# In CASA
flagdata(vis='SNR_G55_10s.ms', mode='shadow', tolerance=0.0, flagbackup=False)
In this particular observation, there does not appear to be much data affected by shadowing, as can be seen in the logger report. One reason why this may be the case, is the antennas were pointed at sources high in elevation.
Zero-Amplitude Data
In addition to shadowing, there may be times during which the correlator writes out pure zero-valued data. In order to remove this bad data, we run flagdata to remove any pure zeroes:
# In CASA
flagdata(vis='SNR_G55_10s.ms', mode='clip', clipzeros=True, flagbackup=False)
Inspecting the logger output which is generated by flagdata shows that there is a small quantity of zero-valued data (4.6%) present in this MS.
Quacking
Now we can utilize the flagdata task one more time in order to run it in quaking mode.
It's common for the array to "settle down" at the start of a scan. Quacking is used to remove data at scan boundaries, and it can apply the same edit to all scans for all baselines.
# In CAS
flagdata(vis='SNR_G55_10s.ms', mode='quack', quackinterval=5.0, quackmode='beg', flagbackup=False)
- quackmode='beg' : Data from the start of each scan will be flagged.
- quackinterval=5.0: Flag the first 5 seconds of every scan.
Backup Data - Flagmanager
Flags can be backed up in a file MS.flagversions that is related to the MS. For our MS the related flag backups are stored in 'SNR_G55_10s.ms.flagversions'.
Note: flags that are applied to the data are contained in the MS. MS.flagversions only contains backup flags that can be restored if required.
Most flagging tasks, like flagdata offer a flagbackup parameter, that controls whether or not the current flags are being backed up in MS.flagversions before new (additional) flags will be applied.
Backup flags can also be manually saved to, or restored from MS.flagversions using the flagmanager task.
Now that we've applied online flags, clipped zero amplitude data, and removed shadowed data, we will create a backup of the flags in such a way, giving it the label 'after_priori_flagging':
# In CASA
flagmanager(vis='SNR_G55_10s.ms', mode='save', versionname='after_priori_flagging')
From here on forward, if we make a mistake, we can always revert back to this version of the MS by setting mode='restore', and providing the version name we want to restore back to.
Hanning-Smoothing
Strong RFI sources can give rise to the Gibbs phenomenon. This is seen by 'ringing', a zig-zag pattern across the channels that neighbor the strong, usually narrow RFI. To remedy this ringing across the frequency channels, we can employ the Hanning smoothing algorithm via the 'hanningsmooth2' task (an implementation based on mstransform. Hanning smoothing applies a triangle kernel across the pattern which reduces the ringing and thus reduces the number of channels that are affected by RFI. This smoothing procedure, however, will also decrease the spectral resolution by a factor of two.
The task allows for the creation of a new MS, or to directly operate on the requested column. For this tutorial, we will be directly operating on the data column and creating a new MS. Note that hanning-smoothing will remove amplitude spikes, it is therefore not recommended for spectral analysis related science, such as HI. Also note that hanning smoothing cannot be reversed once you apply it to your data.
Let's create before and after images with the plotms task, to see the effects of hanning-smoothing the data.
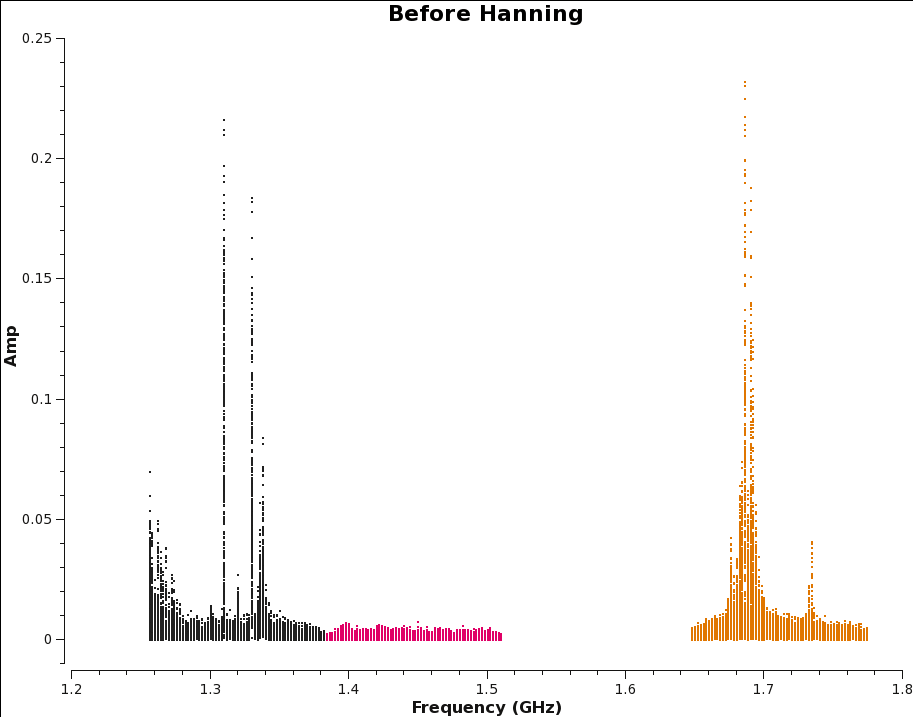
# In CASA
plotms(vis='SNR_G55_10s.ms', scan='190', antenna='ea24', spw='0~2',
xaxis='freq', yaxis='amp', coloraxis='spw', title='Before Hanning',
correlation='RR,LL', plotrange=[1.2,1.8,-0.01,0.25],
plotfile='amp_v_freq.beforeHanning.png')
hanningsmooth2(vis='SNR_G55_10s.ms', outputvis='SNR_G55_10s-hanning.ms', datacolumn='data')
plotms(vis='SNR_G55_10s-hanning.ms', scan='190', antenna='ea24', spw='0~2',
xaxis='freq', yaxis='amp', coloraxis='spw', title='After Hanning',
correlation='RR,LL', plotrange=[1.2,1.8,-0.01,0.25],
plotfile='amp_v_freq.afterHanning.png')
We can compare the generated plots and take notice that single channel RFI has spread into three channels, but it has also removed some of the worst RFI. This spreading of RFI to other channels will ultimately result in a little more data being flagged.
Automatic RFI excision
Now that we're done with priori flagging, we can move on to removing some of the RFI present with auto-flagging algorithms used within flagdata. We will employ two CASA tasks, flagdata (tfcrop) and flagdata (rflag). For further details on the two algorithm based tasks we will employ, please see the presentation given at the 5th VLA Data Reduction Workshop by Urvashi Rau.
TFcrop
flagdata (tfcrop) is an algorithm that detects outliers in the 2D time-frequency plane, and can operate on un-calibrated data (non bandpass-corrected). Tfcrop will iterate through chunks of time, and undergo several steps in order to find and excise different types of RFI. (CASA Cookbook 3.4.2.7)
Step 1: Detect short-duration RFI spikes (narrow-band and broad-band).
Step 2: Search for time-persistent RFI.
Step 3: Search for time-persistent, narrow-band RFI.
Step 4: Search for low-level wings of very strong RFI.
More details on the algorithm steps can be found on this webpage.
We will apply the auto-flagging tfcrop algorithm for each spectral window. We will walk through the first spw and then include the rest with the same command. Let's first plot the corrected data for scan 190, with all spectral windows, so we can compare the data before and after tfcrop.
# In CASA
plotms(vis='SNR_G55_10s-hanning.ms', scan='190', antenna='ea24', xaxis='freq', iteraxis='scan', yaxis='amp',
ydatacolumn='data', plotfile='amp_v_freq_before_tfcrop.png', title='Before TFcrop',
correlation='RR,LL', coloraxis='spw', plotrange=[1.2,2,-0.01,0.25])
Following are a set of flagdata commands which have been found to work reasonably well with these data. Please take some time to play with the parameters and the plotting capabilities. Since these runs set display='both' and action='calculate', the flags are displayed but not actually written to the MS. This allows one to try different sets of parameters before actually applying the flags to the data.
Some representative plots are also displayed. Each column displays an individual polarization product; since we're using all four polarizations, from left to right are RR, RL, LR, and LL. The first row shows the data with current flags applied, and the second includes the flags generated by flagdata. The x-axis is channel number (the spectral window ID is displayed in the top title) and the y-axis of the first two rows is all integrations included in a time "chunk", set by the ntime parameter. These are the data considered by the tfcrop algorithm during its flagging process, and changes in ntime will have some (relatively small) affect on what data are flagged.
Each plot page displays data for a single baseline and time chunk. The buttons at the bottom allow one to step through baseline (backward as well as forward), spw, scan, and field; "Stop Display" will continue the flagging operation without the GUI, and "Quit" aborts the run.
spw 0
# In CASA
flagdata(vis='SNR_G55_10s-hanning.ms', mode='tfcrop', spw='0',
datacolumn='data', action='calculate',
display='both', flagbackup=False)
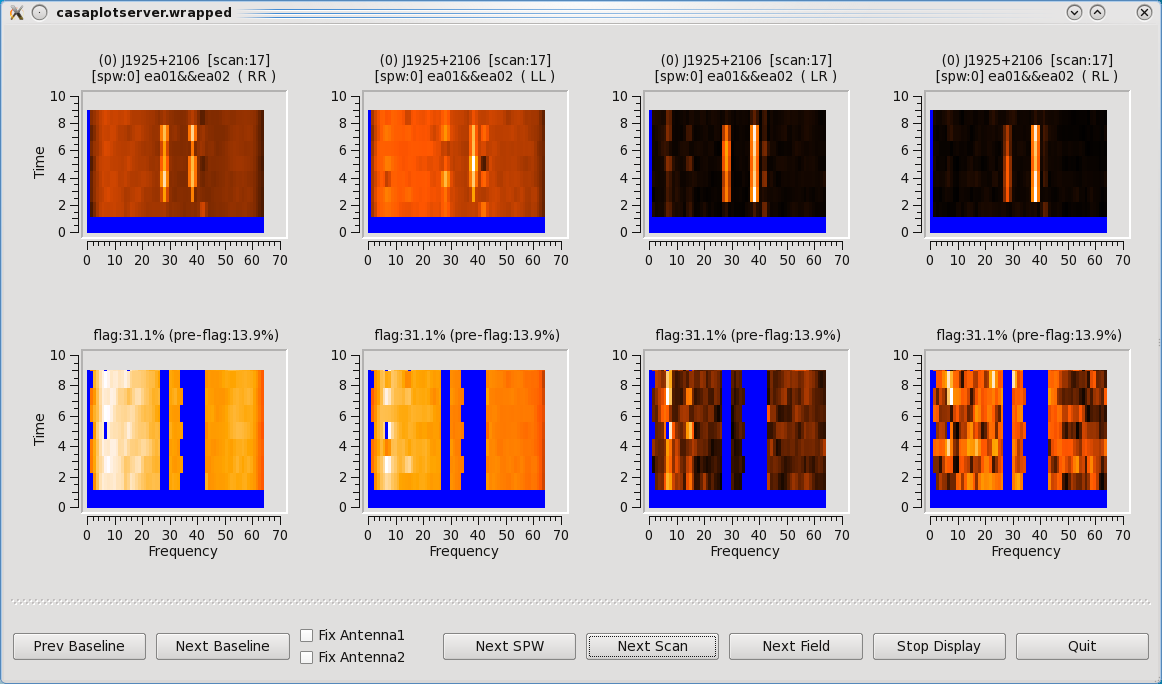
As we iterate through the different scans and baselines, we can see the flagging we can apply, represented by the blue areas. Click on the "Quit" button on the bottom right corner. Let's now apply these flags by changing the action parameter.
# In CASA
flagdata(vis='SNR_G55_10s-hanning.ms', mode='tfcrop', spw='0',
datacolumn='data', action='apply',
display='', flagbackup=False)
The logger will report the percentage of flagged data in the table selection. We can now apply the tfcrop algorithm to the remaining spectral windows.
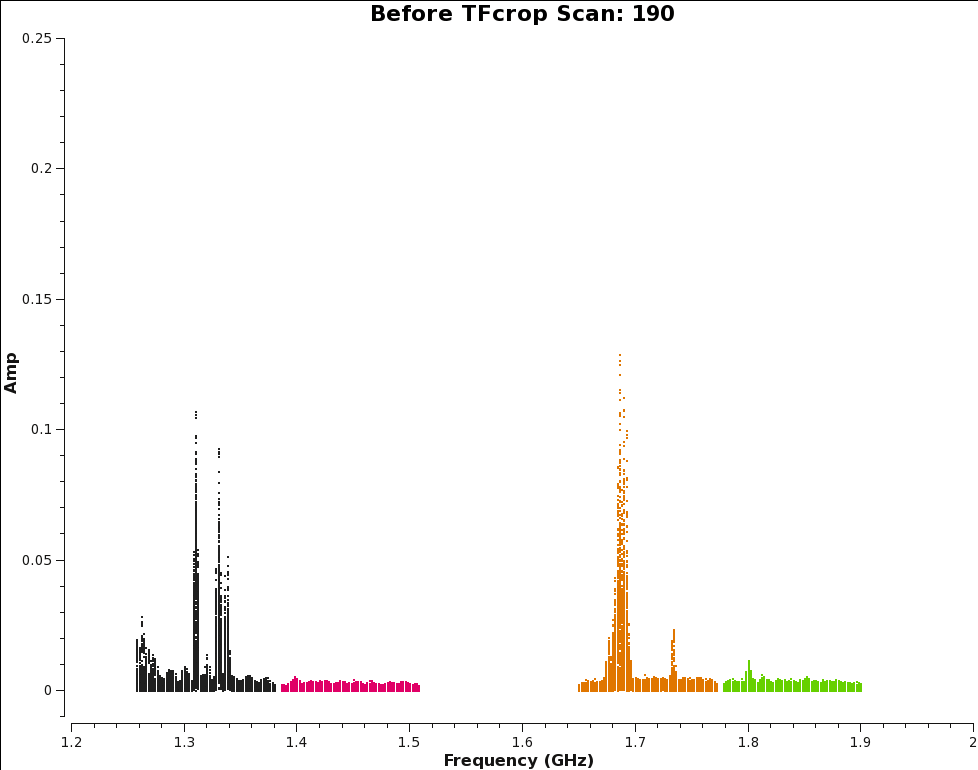
spw 1, 2, 3
# In CASA
flagdata(vis='SNR_G55_10s-hanning.ms', mode='tfcrop', spw='1~3',
datacolumn='data', action='apply',
display='both', flagbackup=False)
Iterate through several scans and baselines, and once you're ready to apply the flags, click on "Stop Display". After tfcrop has gone through and flagged some of the worst RFI, we can inspect the log report and take note of how much has been flagged.
We can also use plotms to review the effects of using tfcrop and compare the image to the one created before applying tfcrop. We can see great improvements, especially for spectral window 0 and 2, which had some of the worst RFI.
# In CASA
plotms(vis='SNR_G55_10s-hanning.ms', scan='190', antenna='ea24', xaxis='freq', iteraxis='scan', yaxis='amp',
ydatacolumn='data', plotfile='amp_v_freq_after_tfcrop.png', title='After TFcrop',
correlation='RR,LL', coloraxis='spw', plotrange=[1.2,2,-0.01,0.25])
We now calculate the amount of flagged data so far in our Measurement Set by using the flagdata task with mode='summary', and assign the returned python dictionary to the variable 'flagInfo'. We then parse the dictionary to print some of the flagging statistics.
# In CASA
flagInfo = flagdata(vis='SNR_G55_10s-hanning.ms', mode='summary')
print("\n %2.1f%% of G55.7+3.4, %2.1f%% of 3C147, and %2.1f%% of J1925+2106 are flagged. \n" %
(100.0 * flagInfo['field']['G55.7+3.4']['flagged'] / flagInfo['field']['G55.7+3.4']['total'],
100.0 * flagInfo['field']['0542+498=3C147']['flagged'] / flagInfo['field']['0542+498=3C147']['total'],
100.0 * flagInfo['field']['J1925+2106']['flagged'] / flagInfo['field']['J1925+2106']['total']))
print("Spectral windows are flagged as follows:")
for spw in range(0,4):
print("SPW %s: %2.1f%%" % (spw, 100.0 * flagInfo['spw'][str(spw)]['flagged'] / flagInfo['spw'][str(spw)]['total']))
The results indicate we have flagged a little over 26% of G55.7+3.4. We can now move on to the other auto-flagging algorithm, rflag.
RFlag
In order to get the best possible result from the automatic RFI excision with flagdata's mode 'rflag', we will first apply bandpass calibration to the MS. Since the RFI is time-variable, using the phase calibration source to make an average bandpass over the entire observation will mitigate the amount of RFI present in the calculated bandpass. (For the final calibration, we will use the designated bandpass source 3C147; however, since this object was only observed in the last set of scans, it doesn't sample the time variability and would not provide a good average bandpass.)
Since there are likely to be gain variations over the course of the observation, we will run gaincal to solve for an initial set of antenna-based phases over a narrow range of channels. Those solutions will be applied to the data when the bandpass solutions are determined. While amplitude variations will have little effect on the bandpass solutions, it is important to solve for these phase variations with sufficient time resolution to prevent decorrelation when vector averaging the data in computing the bandpass solutions.
In order to choose a narrow range of channels for each spectral window which are relatively RFI-free over the course of the observation, we can look at the data with plotms. Note that it's important to only solve for phase using a narrow channel range, since an antenna-specific delay will cause the phase to vary with respect to frequency over the spectral window, perhaps by a substantial amount.
# In CASA
plotms(vis='SNR_G55_10s-hanning.ms', scan='42,65,88,11,134,157', antenna='ea24',
xaxis='channel', yaxis='amp', iteraxis='spw', yselfscale=True, correlation='RR,LL')
- yselfscale=True: sets the y-scaling to be for the currently displayed spectral window, since some spectral windows have much worse RFI and will skew the scale for others.
Looking at these plots, we can choose appropriate channel ranges for each SPW:
SPW 0: 20-24 SPW 1: 49-52 SPW 2: 38-41 SPW 3: 41-44
Using these channel ranges, we run gaincal to calculate phase-only solutions that will be used as input during our initial bandpass calibration. Remember - the calibration tables we are creating now are so that we can use an automatic RFI flagging algorithm. Our final calibration tables will be generated later, after automated flagging. Here are the inputs for our initial pre-bandpass phase calibration:
# In CASA
gaincal(vis='SNR_G55_10s-hanning.ms', caltable='SNR_G55_10s-hanning.initPh', field='J1925+2106', solint='int',
spw='0:20~24,1:49~52,2:38~41,3:41~44', refant='ea24', minblperant=3,
minsnr=3.0, calmode='p')
- caltable='SNR_G55_10s-hanning.initPh': this is the output calibration table that will be written.
- field='J1925+2106': this is the phase calibrator we will use to calibrate the phases.
- solint='int': we request a solution for each 10-second integration.
- pw='0:20~24,1:49~52,2:38~41,3:41~44': note the syntax of this selection: a ":" is used to separate the SPW from channel selection, and "~" is used to indicate an inclusive range.
- refant='ea24': we have chosen ea24 as the reference antenna after inspecting the antenna position diagram (see above). It is relatively close to, but not directly in, the center of the array, which could be important in D-configuration, since you don't want the reference antenna to have a high probability of being shadowed by nearby antennas.
- minblperant=3: the minimum number of baselines which must be present to attempt a phase solution.
- minsnr=3.0: the minimum signal-to-noise a solution must have to be considered acceptable. Note that solutions which fail this test will cause these data to be flagged downstream of this calibration step.
- calmode='p': perform phase-only solutions.
Note that a number of solutions do not pass the requirements of the minimum 3 baselines (generating the terminal message "Insufficient unflagged antennas to proceed with this solve.") or minimum signal-to-noise ratio (outputting "n of x solutions rejected due to SNR being less than 3 ..."). The logger output indicates 324 (320 for spw0) solutions succeeded, out of 387 attempted.
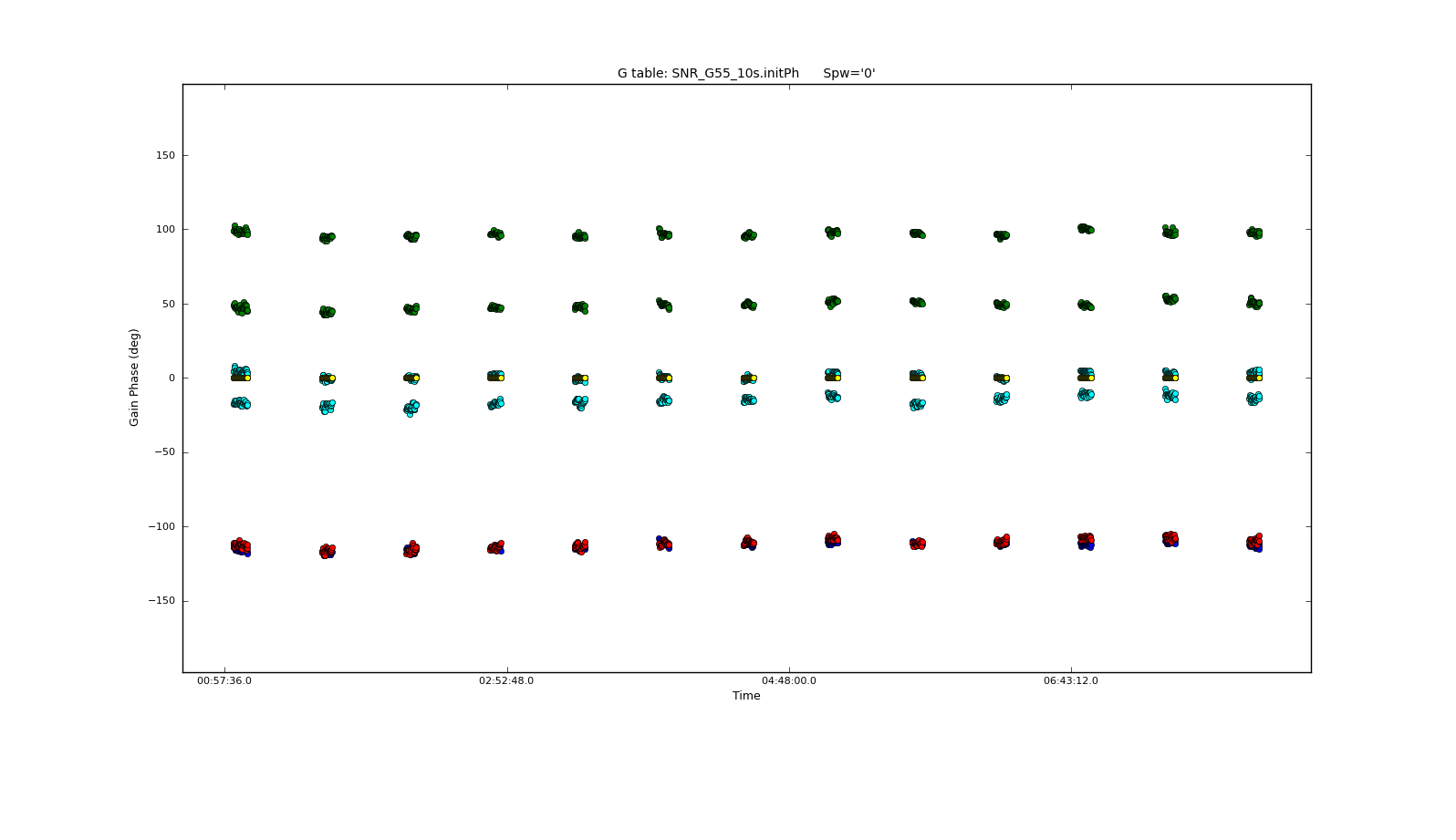
It's always a good idea to inspect the resulting calibration table with plotcal. We will first iterate over antennas for spectral window 0.
# In CASA
plotcal(caltable='SNR_G55_10s-hanning.initPh', xaxis='time', yaxis='phase', iteration='antenna',
spw='0', plotrange=[-1,-1,-180,180])
We can see that the phases do not change much over the course of the observation. Let's now inspect the other spectral windows for a few of the antennas.
# In CASA
plotcal(caltable='SNR_G55_10s-hanning.initPh', xaxis='time', yaxis='phase', iteration='spw',
antenna='ea01,ea05,ea10,ea24', plotrange=[-1,-1,-180,180])
Now that we've determined our plots look fairly reasonable, we will create a time-averaged bandpass solutions for the phase calibration source using the bandpass task.
# In CASA
bandpass(vis='SNR_G55_10s-hanning.ms', caltable='SNR_G55_10s-hanning.initBP', field='J1925+2106', solint='inf', combine='scan',
refant='ea24', minblperant=3, minsnr=10.0, gaintable='SNR_G55_10s-hanning.initPh',
interp='nearest', solnorm=False)
- solint='inf', combine='scan': the solution interval of 'inf' will automatically break by scans; this requests that the solution intervals be combined over scans, so that we will get one solution per antenna.
- gaintable= 'SNR_G55_10s-hanning.initPh': we will pre-apply the initial phase solutions.
- interp='nearest': by default, bandpass will use linear interpolation for pre-applied calibration. However, we want the nearest phase solution to be used for a given time.
Again, we can see that a number of solutions have been rejected by our choice of minsnr.
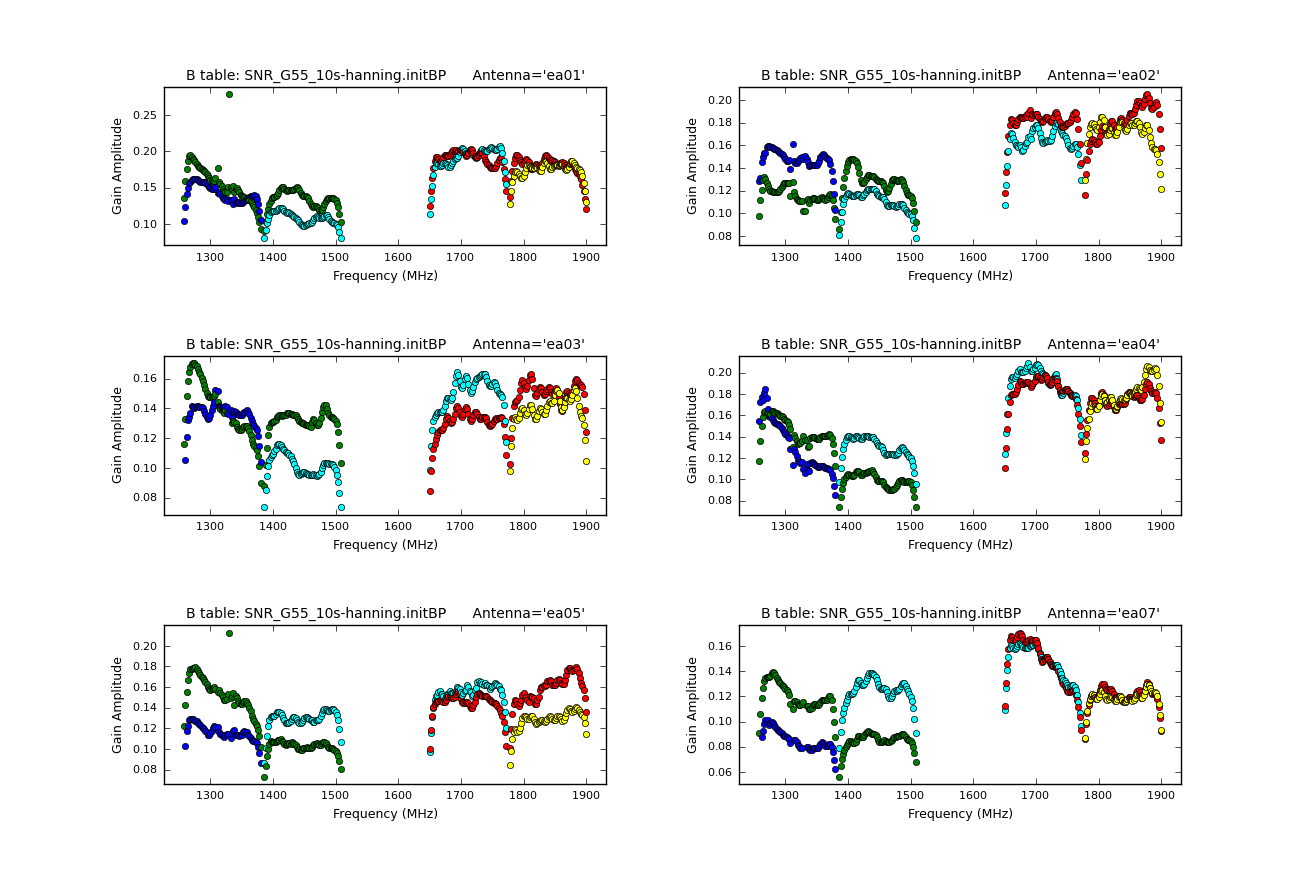
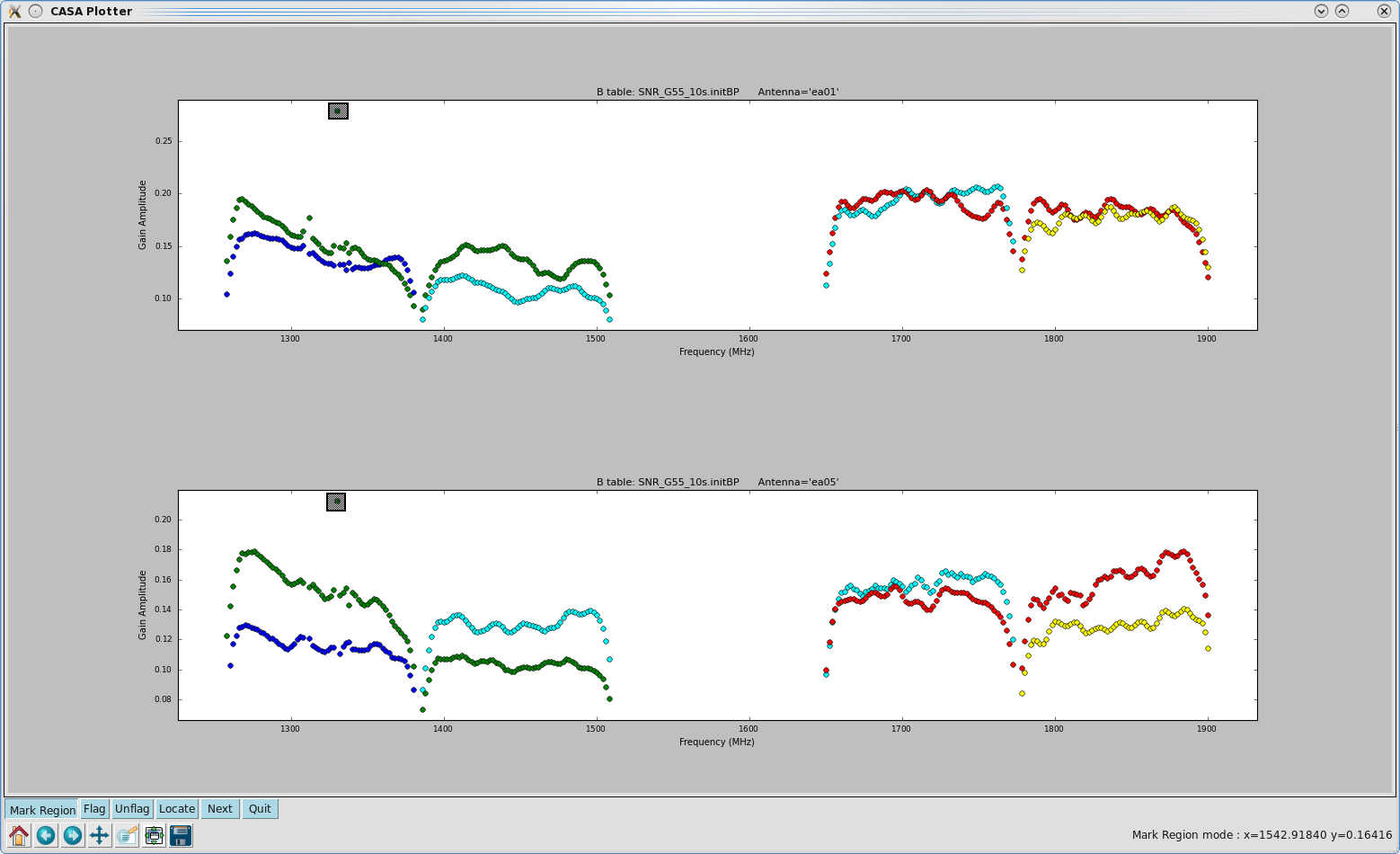
Let us now inspect the resulting bandpass plots with plotcal.
# In CASA
plotcal(caltable='SNR_G55_10s-hanning.initBP', xaxis='freq', yaxis='amp',
iteration='antenna', subplot=331)
- subplot=331: displays 3x3 plots per screen
We notice that antenna's ea01 and ea05 have a point that is offset from the rest. Let's plot just these two antennas, locate the point on the plot, and flag it interactively through plotcal. Please note that interactive flagging within plotcal will not create a backup, therefore it may be wise to use flagmanager before doing so.
# In CASA
plotcal(caltable='SNR_G55_10s-hanning.initBP', antenna='ea01,ea05', xaxis='freq', yaxis='amp',
iteration='antenna', subplot=211)
We will highlight the points by clicking on the "Mark Region" button, drawing boxes over the points, and clicking on the "Flag" button. Before doing this, one could also get information on the points by clicking on the "Locate" button, which will display details about the highlighted regions. After having flagged the offset points, your plots should update.
We will now apply the bandpass calibration table using applycal.
# In CASA
applycal(vis='SNR_G55_10s-hanning.ms', gaintable='SNR_G55_10s-hanning.initBP', calwt=False)
This operation will flag data that correspond to flagged solutions, so applycal makes a backup version of the flags prior to operating on the data. Note that running applycal might take a little while.
Now that we have bandpass-corrected data with some RFI flagged out, we will run flagdata in rflag mode (CASA Cookbook 3.4.2.8). RFlag, like TFcrop, is an autoflag algorithm which uses a sliding window statistical filter. Data is iterated through in chunks of time, where statistics are accumulated, and thresholds calculated.
Additional information on the algorithm used in rflag, as well as the statistical details that are undertaken can be found on this webpage (sections 2.1.7).
We will use flagdata with mode='rflag', and action='calculate' to first review the amount of data to be flagged. We will also change the datacolumn parameter to 'corrected', since we've applied the bandpass corrections to the MS, and in the process, created the corrected_data column in the MS table.
spw 0
First, let's create a plot of our corrected data before we apply RFlag.
# In CASA
plotms(vis='SNR_G55_10s-hanning.ms', scan='190' , antenna='ea24',
xaxis='freq', yaxis='amp', ydatacolumn='corrected', coloraxis='spw', title='Before RFlag',
correlation='RR,LL', plotfile='amp_v_freq.beforeRFlag.png')
flagdata(vis='SNR_G55_10s-hanning.ms', mode='rflag', spw='0', datacolumn='corrected',
action='calculate', display='both', flagbackup=False)
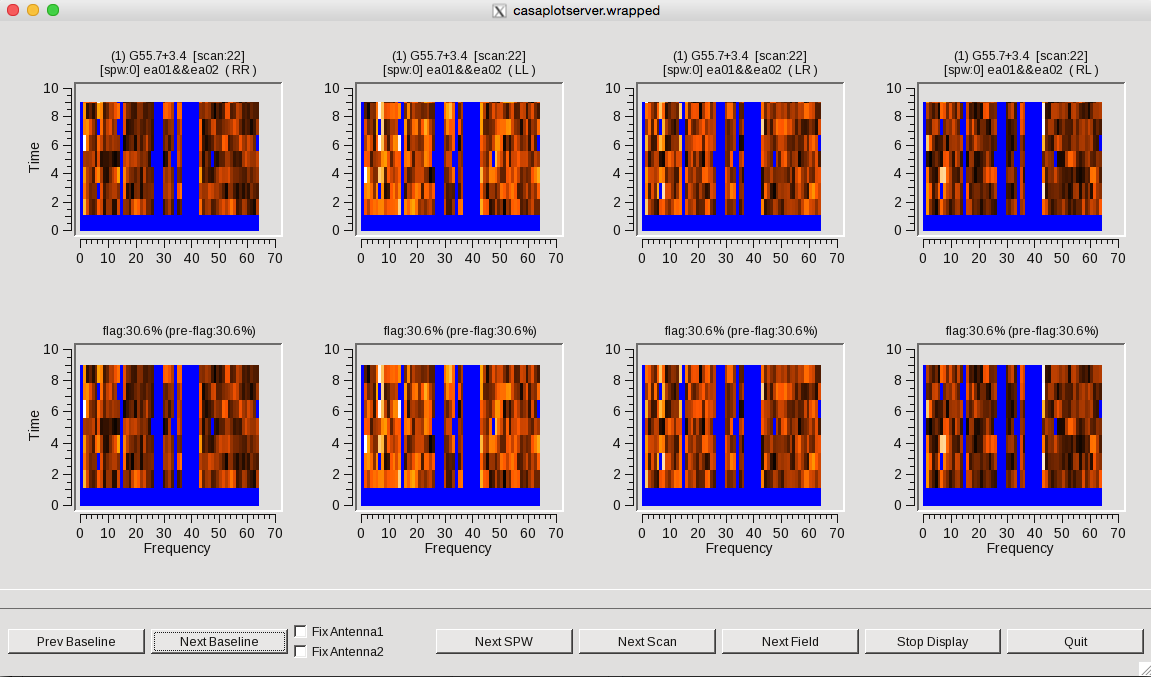
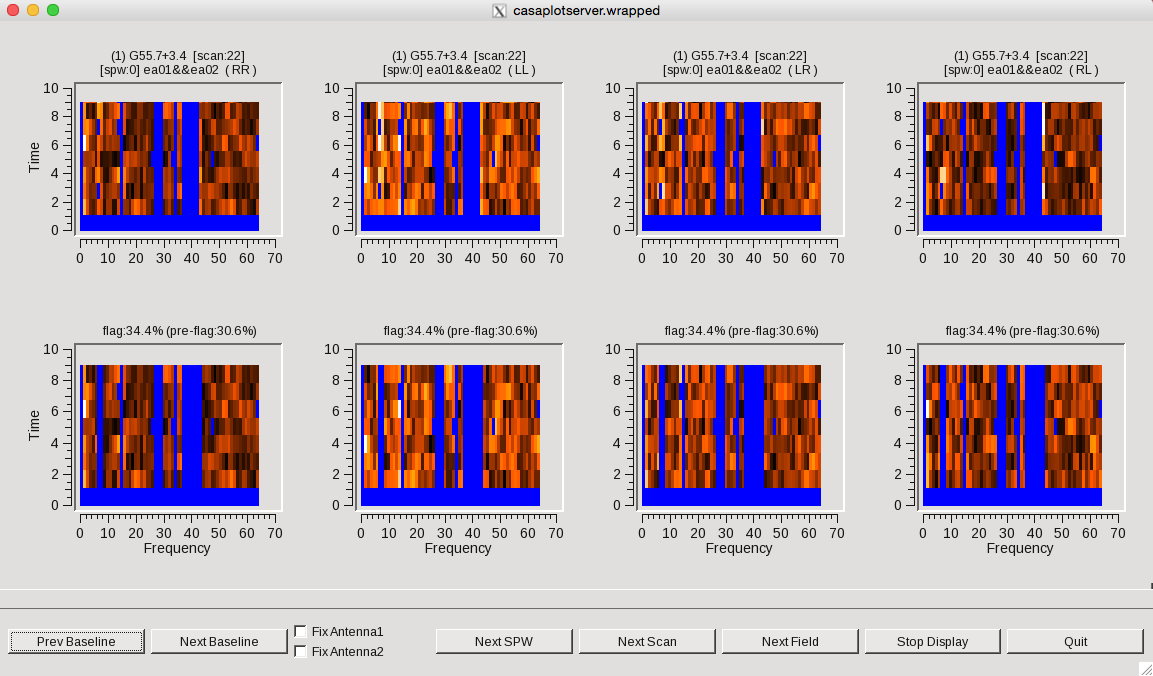
We can see that a lot of RFI is being missed by the default timedevscale and freqdevscale parameters of 5.0. Since we know spw 0 has lots of RFI, we will change the values to 1.5 and apply them. Note that we can always decide that this still isn't good enough and click on "Quit" to change our parameter values.
# In CASA
flagdata(vis='SNR_G55_10s-hanning.ms', mode='rflag', spw='0', datacolumn='corrected',
freqdevscale=1.5, timedevscale=1.5, action='apply', display='both', flagbackup=False)
Although RFlag has done a pretty good job of finding the bad data, some still remains. One way to excise the remaining bad data is to use the mode='extend' feature in flagdata, which can extend flags along a chosen axis and removes 'islands' of small data patches in the midst of flagged data. First, we will extend the flags across polarization, so if any one polarization is flagged, all data for that time / channel will be flagged:
# In CASA
flagdata(vis='SNR_G55_10s-hanning.ms', mode='extend', spw='0', extendpols=True,
action='apply', display='', flagbackup=False)
Now, we will extend the flags in time and frequency, using the "growtime" and "growfreq" parameters. For the data here, the rflag algorithm seems most likely to miss RFI which should be flagged along more of the time axis, so we will try with growtime=50.0, which will flag all data for a given channel if more than 50% of that channel's time is already flagged, and growfreq=90.0, which will flag the entire spectrum for an integration if more than 90% of the channels in that integration are already flagged.
# In CASA
flagdata(vis='SNR_G55_10s-hanning.ms', mode='extend', spw='0', growtime=50.0,
growfreq=90.0, action='apply', display='', flagbackup=False)
spw 1, 2, and 3
Now, let's work on SPW's 1,2, and 3. We've chosen display='both', in order to first review the flags, and decide if too much or too litle is being flagged. We can always click on 'Quit', and decide to change the freqdevscale and timedevscale parameters, as we did with spectral window 0. Note that spw 2 has these parameters lower than spw 1/3, as it contains more RFI. To allow the flagging to continue, click on "Stop Display".
# In CASA
flagdata(vis='SNR_G55_10s-hanning.ms', mode='rflag', spw='1,3', datacolumn='corrected',
freqdevscale=4.0, timedevscale=4.0, action='apply', display='both', flagbackup=False)
flagdata(vis='SNR_G55_10s-hanning.ms', mode='extend', spw='1,3', extendpols=True,
action='apply', display='', flagbackup=False)
flagdata(vis='SNR_G55_10s-hanning.ms', mode='extend', spw='1,3', growtime=50.0,
growfreq=90.0, action='apply', display='', flagbackup=False)
flagdata(vis='SNR_G55_10s-hanning.ms', mode='rflag', spw='2', datacolumn='corrected',
freqdevscale=2.5, timedevscale=2.5, action='apply', display='both', flagbackup=False)
flagdata(vis='SNR_G55_10s-hanning.ms', mode='extend', spw='2', extendpols=True,
action='apply', display='', flagbackup=False)
flagdata(vis='SNR_G55_10s-hanning.ms', mode='extend', spw='2', growtime=50.0,
growfreq=90.0, action='apply', display='', flagbackup=False)
Let's create an after RFlag plot to see the improvements.
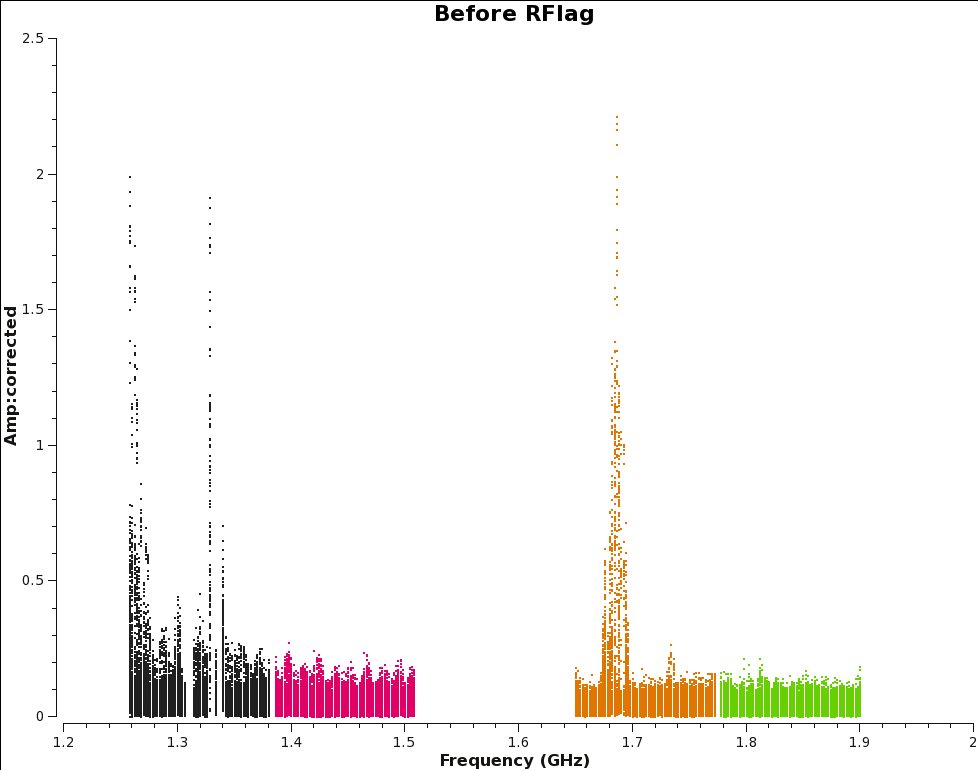
# In CASA
plotms(vis='SNR_G55_10s-hanning.ms', scan='190' , antenna='ea24',
xaxis='freq', yaxis='amp', ydatacolumn='corrected', coloraxis='spw', title='After RFlag',
correlation='RR,LL', plotfile='amp_v_freq.afterRFlag.png', plotrange=[1.2,2,0,2.5])
We will now plot amplitude vs. baseline, and look for outliers which we may be able to flag.
# In CASA
plotms(vis='SNR_G55_10s-hanning.ms', scan='30,75,120,165,190,235,303', xaxis='baseline', yaxis='amp',
ydatacolumn='corrected', iteraxis='scan', correlation='RR,LL', coloraxis = 'baseline')
It appears that there are a several baselines which have consistently higher-amplitude than the others, indicating that they're probably contaminated by RFI.
Use the plotms tools to identify the baselines (see above how to do that). We will flag just a few, ea04&ea16 and ea04&ea21.
# In CASA
flagdata(vis='SNR_G55_10s-hanning.ms', antenna='ea04&ea16', spw='1', flagbackup=False)
flagdata(vis='SNR_G55_10s-hanning.ms', antenna='ea04&ea21', spw='1', flagbackup=False)
Interactive Flagging
After plotting our data, it's obvious that there is still some amount of RFI present. We can use plotms to interactively flag points which look bad. A tutorial on flagging data interactively through plotms can be found here. Please note that flagging data through plotms will not create a backup, so it's important to use the flagmanager before deciding to mark your regions for flagging purposes.
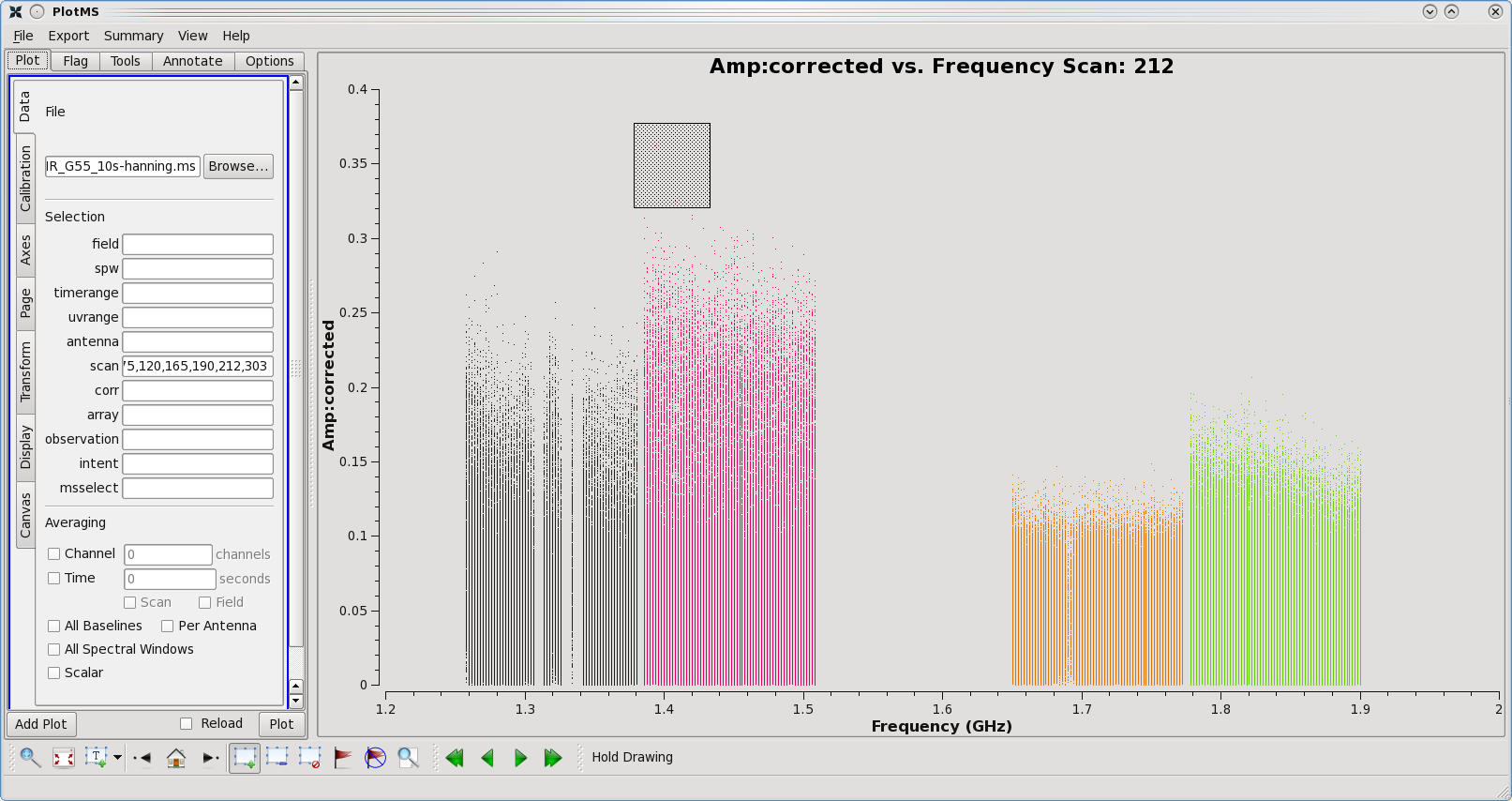
# In CASA
flagmanager(vis='SNR_G55_10s-hanning.ms', mode='save',
versionname='before_interactive_flagging')
plotms(vis='SNR_G55_10s-hanning.ms', scan='30,75,120,165,190,235,303', xaxis='frequency', yaxis='amp',
ydatacolumn='corrected', iteraxis='scan', correlation='RR,LL', coloraxis = 'spw')
Let's iterate through scans and view scan 75. We can see that spectral window 1 has a large amplitude spike. We can use the "mark regions" button (square with green plus sign) to highlight a majority of spike, and the "locate" button (magnifying glass) to give us information on the highlighted region. We will now click on the "flag" button (red flag) and we should see the plot update, having removed the highlighted region. Feel free to flag more data if you'd like.
Flagging Summary & Report
We will now run flagdata in summary mode to inspect how much data we have flagged in total. We can also create a plot of the percentage of flagged data with respect to frequency by setting display='report'. This parameter will also create a plot of the antennas, with the circle size representing the percentage of flagged data per antenna.
# In CASA
flagInfo = flagdata(vis='SNR_G55_10s-hanning.ms', mode='summary', action='calculate', display='report', spwchan=T)
print("\n %2.1f%% of G55.7+3.4, %2.1f%% of 3C147, and %2.1f%% of J1925+2106 are flagged. \n" %
(100.0 * flagInfo['field']['G55.7+3.4']['flagged'] / flagInfo['field']['G55.7+3.4']['total'],
100.0 * flagInfo['field']['0542+498=3C147']['flagged'] / flagInfo['field']['0542+498=3C147']['total'],
100.0 * flagInfo['field']['J1925+2106']['flagged'] / flagInfo['field']['J1925+2106']['total']))
print("Spectral windows are flagged as follows:")
for spw in range(0,4):
print("SPW %s: %2.1f%%" % (spw, 100.0 * flagInfo['spw'][str(spw)]['flagged'] / flagInfo['spw'][str(spw)]['total']))

So, as a result of the flagging, we have sacrificed 36% of the data for G55.7+3.4, and as expected, spectral windows 0 and 2 have the most flagged data.
-- original: Miriam Hartman
--modifications: Lorant Sjouwerman (4.4.0, 2015/07/07)
--modifications: Juergen Ott (4.5.2, 2016/04/13)
--modifications: Jose Salcido (4.5.2, 2016/04/14)
Last checked on CASA Version 4.5.2